Tackling today’s challenge in ultra-low power wearables
The rise of ultra-low power wearables, designed to operate with firmware that is bare-bones and heavily constrained, poses significant challenges. These devices, often screenless, buttonless, or even battery-less, demand efficiency and intelligence far beyond the capabilities of traditional operating systems.
Current solutions fall short in supporting AI-native workloads, handling multi-sensory inputs, and enabling collaborative intelligence, limiting the potential of wearables in constrained environments. A new approach is urgently needed to meet these demands.
Our solution
OmniOS is a groundbreaking AI-native operating system specifically designed for ultra-low power wearables operating under constrained conditions. With a focus on minimal firmware and optimal resource utilization, OmniOS redefines efficiency and functionality.
It leverages advanced technologies such as hetero-accelerator orchestration and data-centric collaborative AI to empower wearables to perform AI-native tasks seamlessly. This innovation enables devices to unlock new functionalities, ensuring they meet the demands of constrained environments while delivering exceptional performance and intelligence.
Key technology enablers
Hetero-accelerator orchestration
efficiently manages diverse computational resources to optimise AI workload execution for ultra-constrained devices
Data-centric collaborative AI
facilitates intelligent data-sharing across connected wearables, boosting performance and capabilities
Cross-service holistic storage management
enhances data handling across multiple devices, ensuring seamless operation in constrained environments
Software-defined thermal management
uses advanced software techniques to maintain optimal performance, even under stringent thermal limitations
OmniOS bridges the gap between constrained firmware environments and the increasing demands of AI-native applications, delivering unmatched efficiency and enabling new possibilities for next-generation wearables.
APA style publications
- Gong, T., Kawsar, F., Min, C. "DEX: Data Channel Extension for Efficient CNN Inference on Tiny AI Accelerators" in Neural Information Processing Systems (NeurIPS), 2024
- Min, C., Acer, U. G., Jang, S., Choi, S., Vasile, D. A., Gong, T., Yi, J., & Kawsar, F. (2024). An AI-Native Runtime for Multi-Wearable Environments. arXiv. https://arxiv.org/abs/2403.17863
Discover more
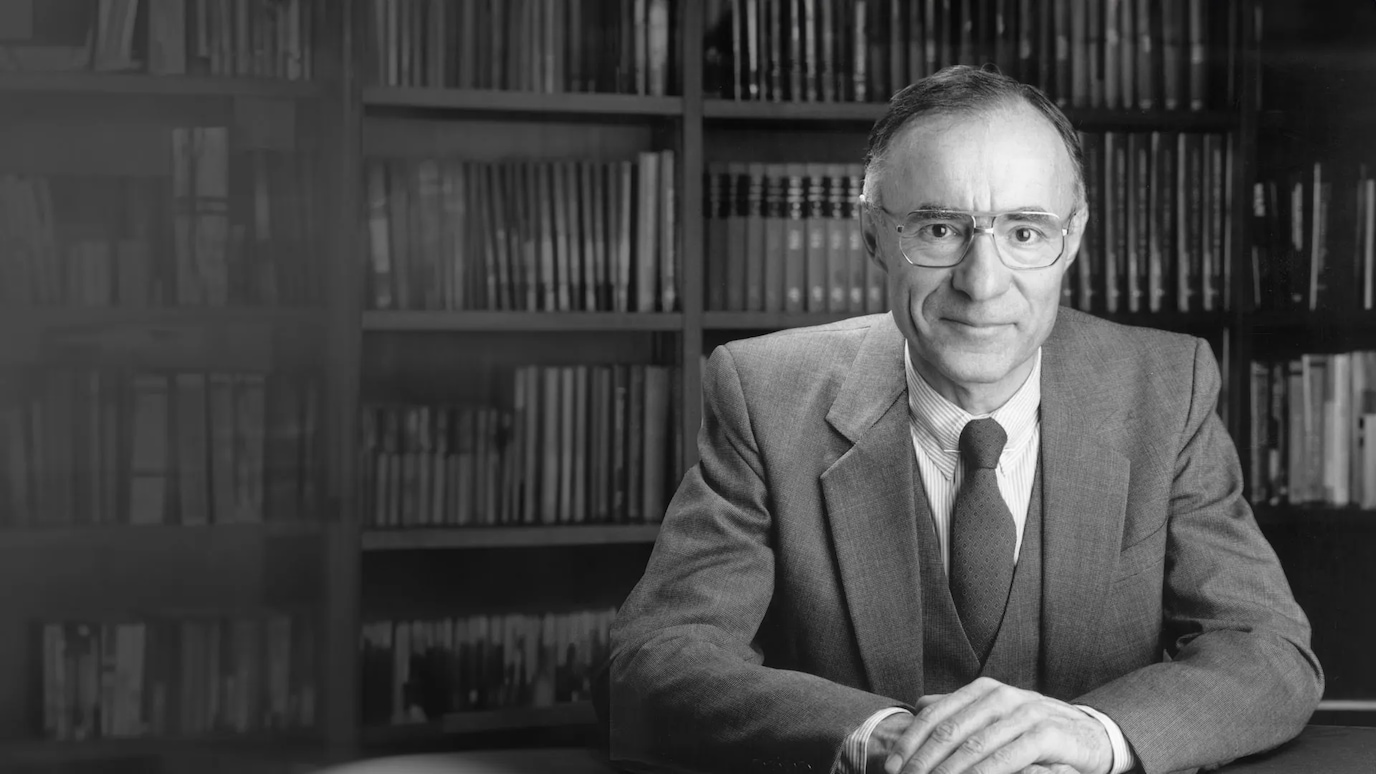
Biographies
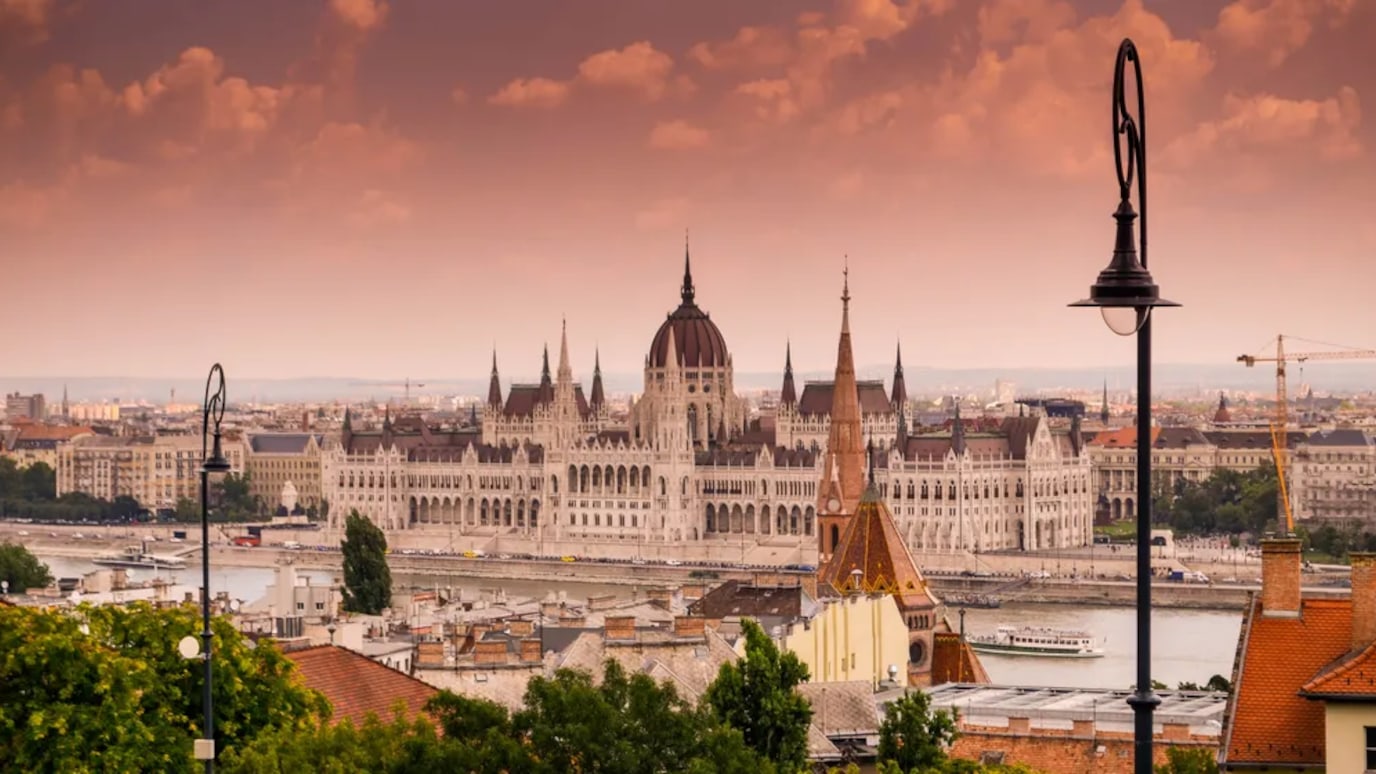
Location
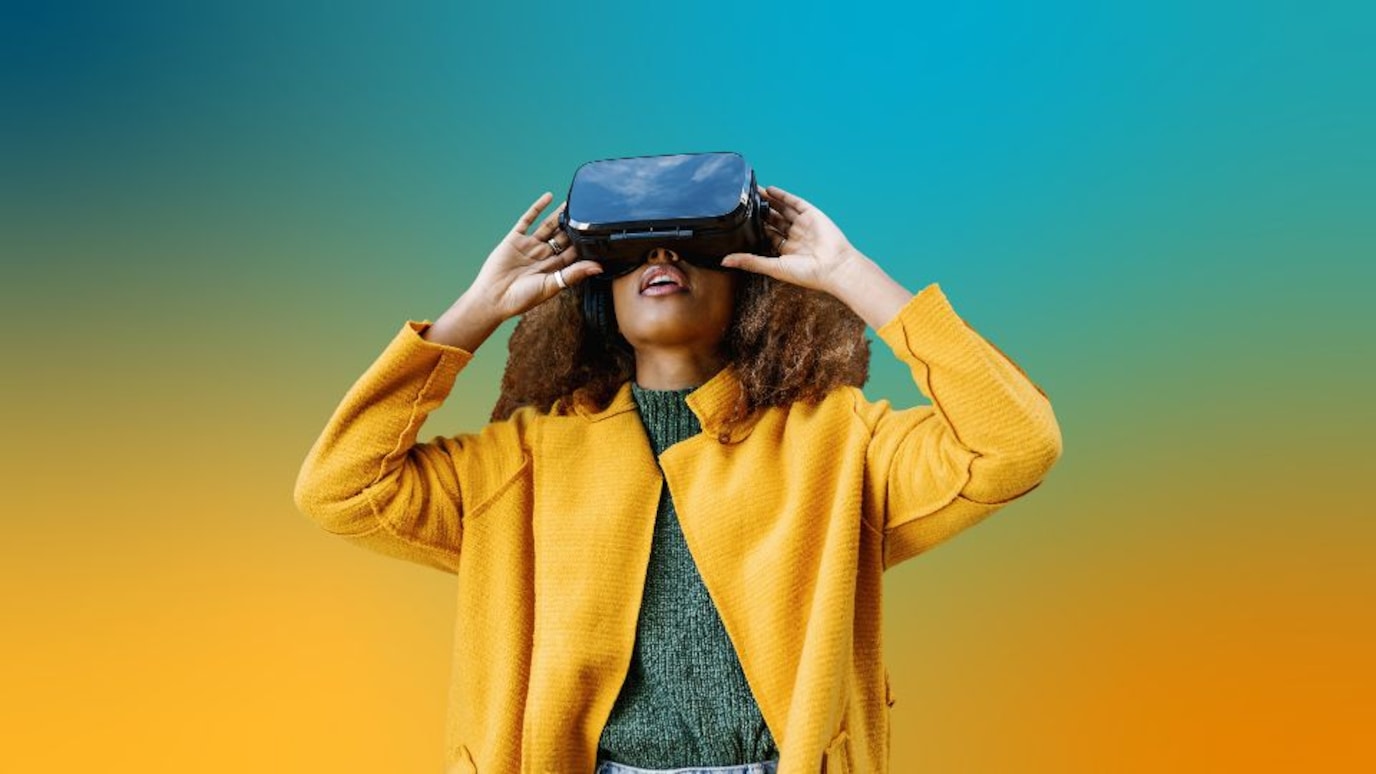
Service
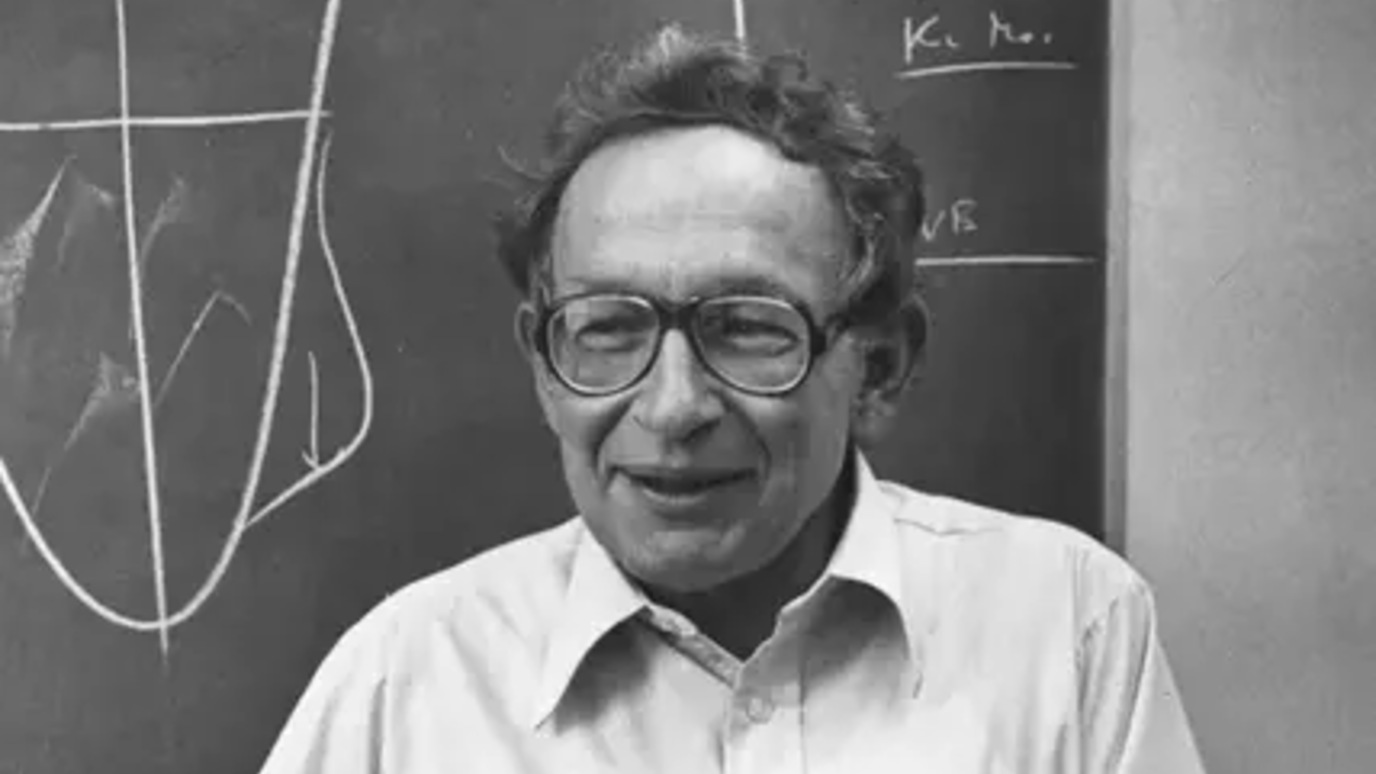
Awards
Please complete the form below.
The form is loading, please wait...
Thank you. We have received your inquiry. Please continue browsing.