Project overview
Robotic arms play a major role in many industrial and home automation applications. Reinforcement learning (RL) has made huge progress in robotic arm manipulation and grasping. While RL has produced attractive results in simulated environments, their real-world feasibility continues to be limited by two major challenges: sample inefficiency and the inability to adapt to another domain or the real world. Our research focuses on addressing these two challenges.
One of our recent studies has focused on re-configurable robots which can potentially have more utility and flexibility for many real-world tasks. Designing a learning agent to operate such robots would require the ability to adapt to different configurations. We focus on robotic arms with multiple rigid links connected by joints. Recent attempts have performed domain adaptation and Sim2Real transfer that provide robustness to variations in robotic arm dynamics and sensor/camera variations. However, there have been no previous attempts to adapt to robotic arms with varying number of links.
We propose an RL agent, with sequence neural networks embedded in the deep neural network, to adapt to robotic arms that have varying number of links. Further, with the additional tool of domain randomization, this agent adapts to different configurations with varying number and length of links and dynamics noise. More details can be found here.
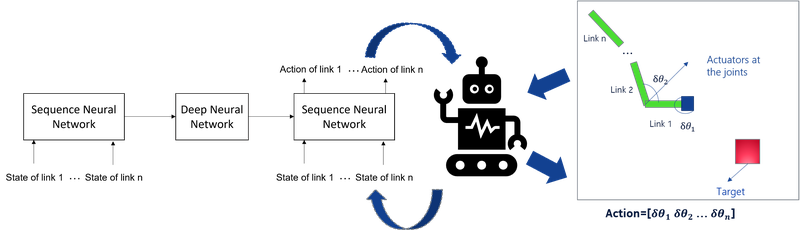
Project members
APA style publications
-
Hosangadi, H.; Kumar, A. R. ”Malleable Agents for Re-Configurable Robotic Manipulators” 2022 ArXiv preprint - arXiv:220.02395 (Link)
Discover more
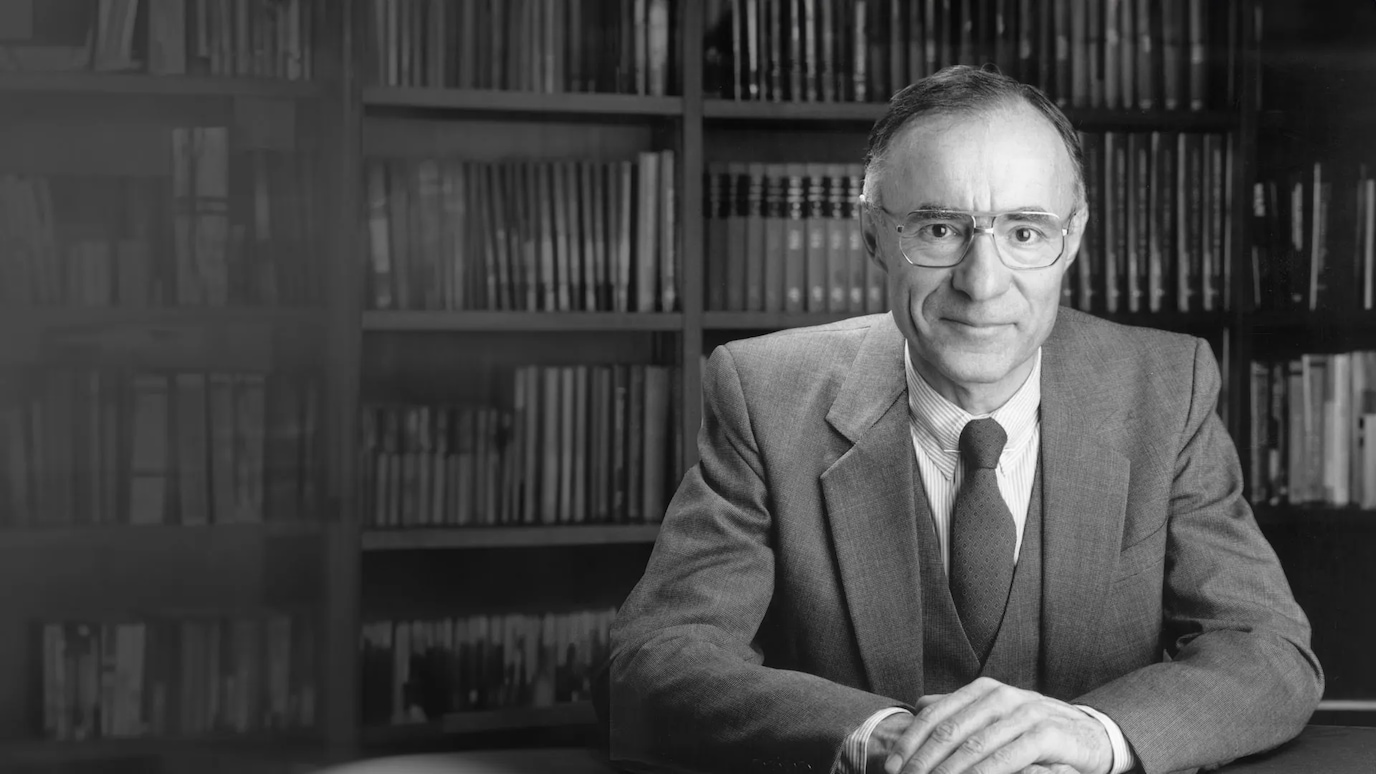
Biographies
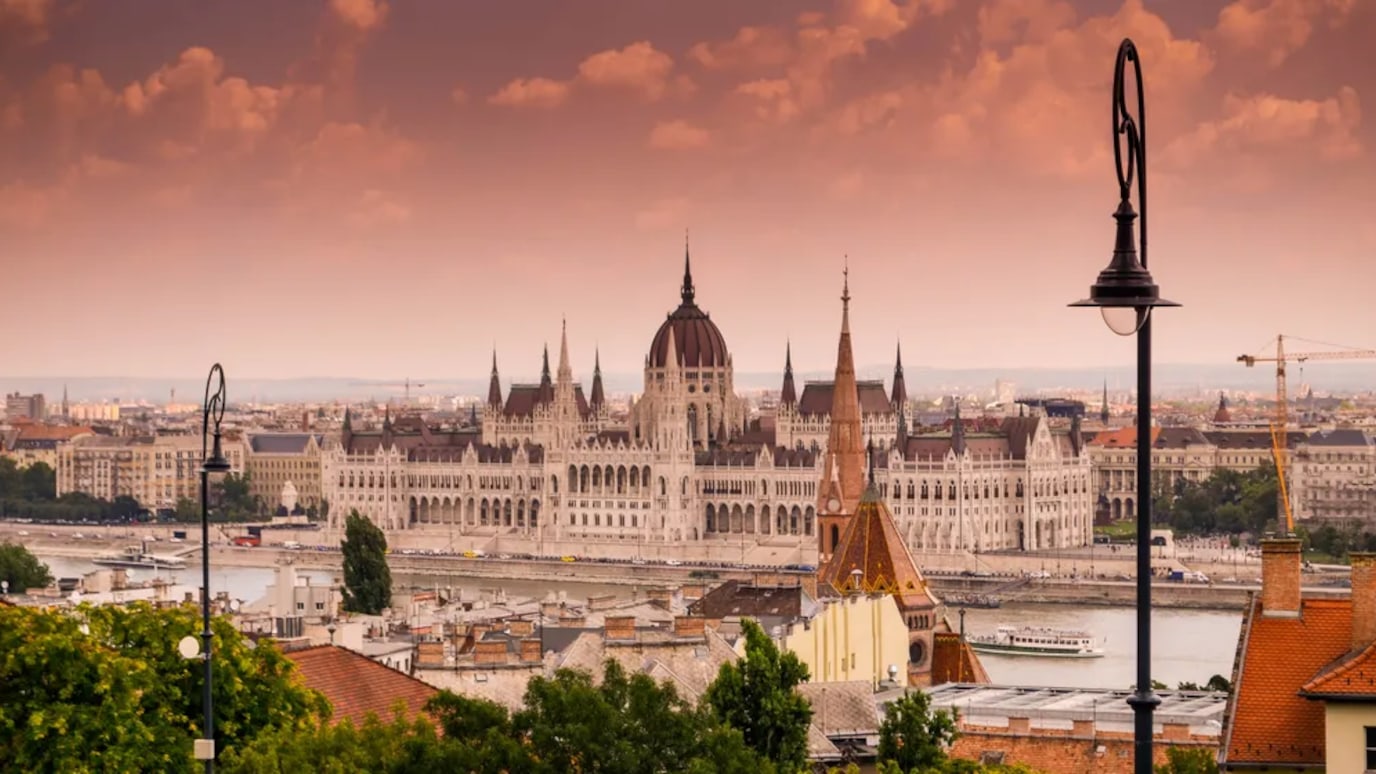
Location
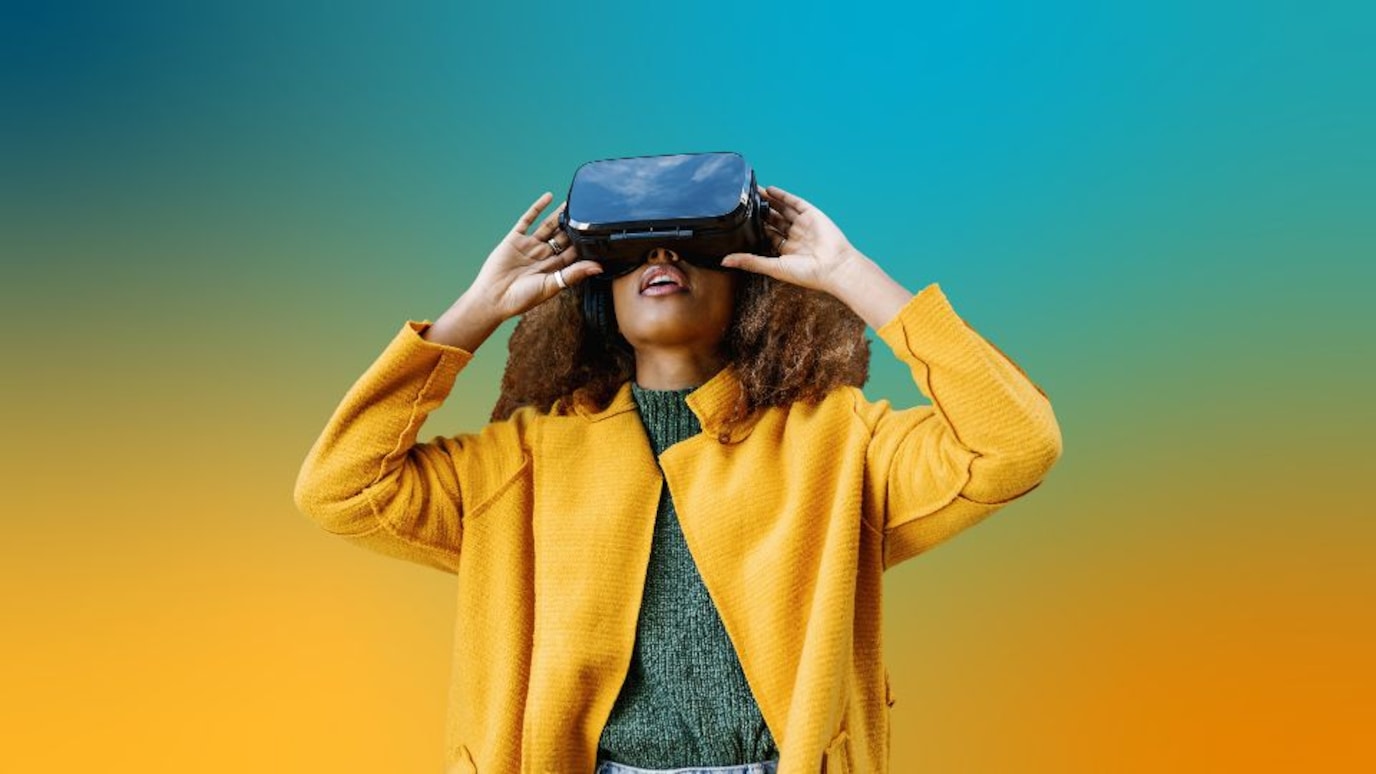
Service
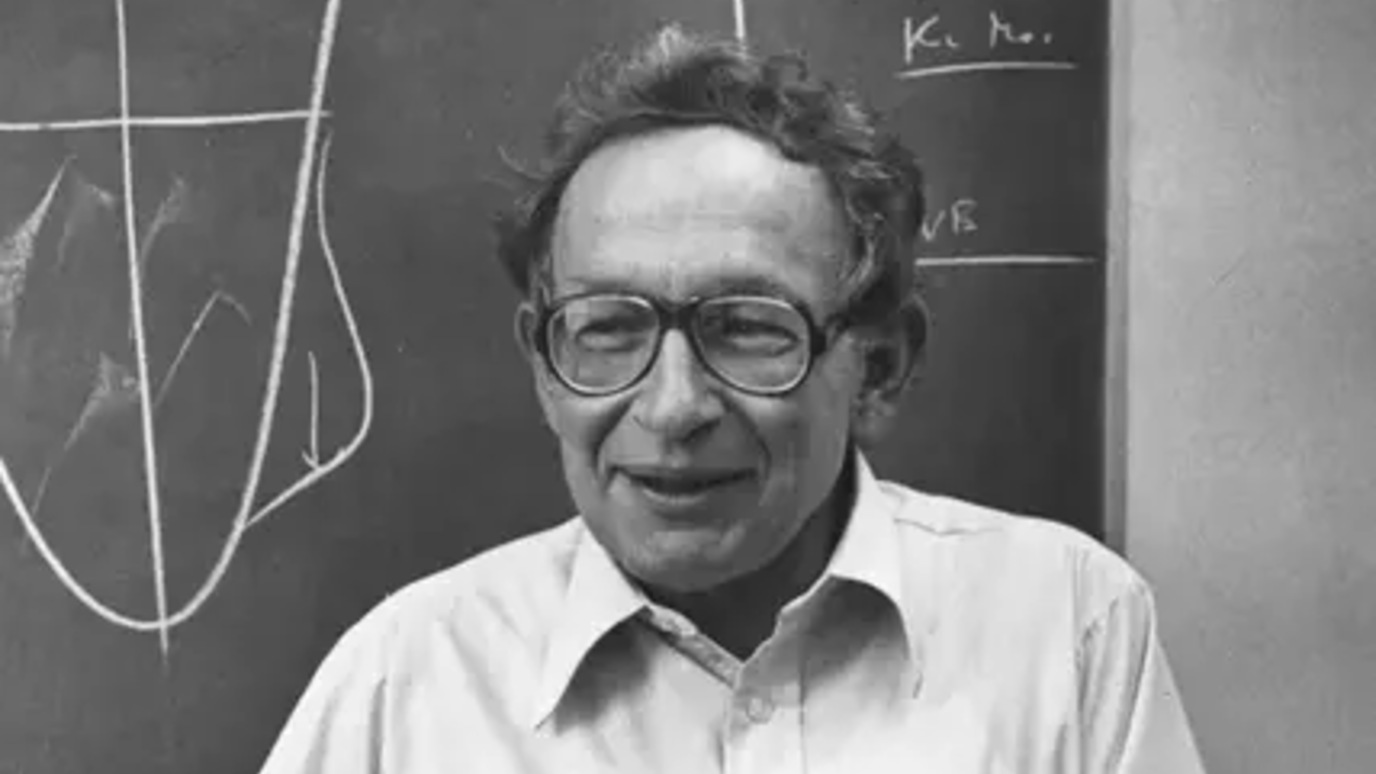
Awards