AI boosts Versatile Video Coding
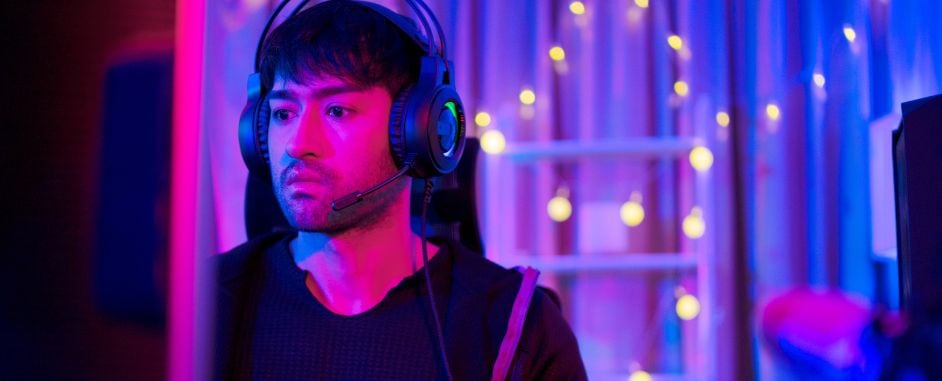
Standard for neural-network post-filtering completed
Versatile Video Coding (VVC), the most efficient video compression standard in the market, is now even better thanks to an artificial intelligence (AI) extension that enhances picture quality and provides functionality. This is a real landmark in the history of video coding standards, as it is arguably the very first time AI is introduced as an integral part of a standard.
What is neural-network-based post-filtering
The recently completed Versatile Supplemental Enhancement Information (VSEI) standard compliments VVC by (among other things) helping a device to enhance image quality after decoding the content.
The VSEI standard defines the required information for tasks beyond traditional video coding, such as adapting decoded video to display. VSEI includes the syntax and semantics of Supplemental Enhancement Information (SEI) messages, which allow decoders to interpret them consistently across different systems.
The latest version of the VSEI standard, which took over two years to develop, has brought AI into video coding standards for the first time. Specifically, it introduces two SEI messages for neural-network-based post-processing: the neural-network post-filter characteristics (NNPFC) SEI message and the neural-network post-filter activation (NNPFA) SEI message. These messages enable the flexible application of neural networks as post-filters, which improves decoded pictures or creates additional interpolated pictures.
The NNPFC SEI message lets an encoder define a neural network for post-processing after decoding. It also specifies the input and output of the neural network and describes its complexity. Additionally, the NNPFC SEI message indicates the intended purpose of the neural network, which can include:
- Enhancing visual quality
- Changing spatial resolution, e.g., from high-definition decoded video to ultra-high definition
- Upsampling picture rate, e.g., from 30 Hz to 60 Hz
- Upsampling bit depth to increase the dynamic range of pixel values
- Colorization to convert monochrome video to full colors.
By implementing neural-network filters as a post-processing step, their utilization can be introduced to services without negatively impacting older systems that lack the ability to execute neural networks.
Improved performance through content adaptation
The VSEI design adapts the neural-network post-filters and their use according to the content, which is why the NNPFC and NNPFA SEI messages are included in the video bitstream instead of relying on generic content-unaware filters in player devices. The adaptation process works as follows:
- The video encoder can define multiple post-filters using NNPFC SEI messages and select the filter that produces the most desirable results for each picture. The chosen filter is indicated through the NNPFA SEI message.
- The neural network can be fine-tuned specifically for the content being encoded. The fine-tuned parameters of the neural network are encoded using the weight update feature of the Neural Network Compression (NNC) standard's recently completed Edition 2 and included in an NNPFC SEI message.
Nokia at the forefront of video coding
Nokia has been among the most active contributors to the NNPFC and NNPFA SEI messages and also holds an editor position in the latest version of the VSEI standard. Moreover, having been one of the key contributors to NNC, Nokia pioneered developing content-adaptive fine-tuning techniques for neural networks used in quality-enhancement post-filters.
Our research findings suggest that neural-network post-filters with a complexity suitable for real-time operation in current consumer products, can deliver a quality enhancement equivalent to approximately an eight percent reduction in bitrate[1]. This gain comes on top of the bitrate reduction of about 50 percent that VVC provides compared to the previous generation of video coding standards. Furthermore, our findings indicate that content-adaptive fine-tuning contributes approximately half of the overall improvement in quality achieved through post-filtering.
Along with other contributors to standardization, Nokia is actively pushing forward AI-based post-filtering technology by enabling its selective use in video file playback and streaming. This technology allows players to choose whether or not to use the recommended neural networks provided by the content creator, and if so which ones.
Furthermore, we are exploring more advanced uses of post-filters, such as cascading multiple filters and applying filters based on specific regions. In the future, the development and adaptation of these technologies will enable a variety of exciting new use cases, such as video enhancement for improved machine vision performance. AI will not only make video look better but also help machines see better!
References
[1] M. Santamaria, R. Yang, F. Cricri, H. Zhang, J. Lainema, R. G. Youvalari, H. R. Tavakoli, and M. M. Hannuksela, "Overfitting multiplier parameters for content-adaptive post-filtering in video coding", Proceedings of 10th European Workshop on Visual Information Processing (EUVIP), Oct. 2022.