Bell Labs Prize winner devises an image-recognition technology powered by light
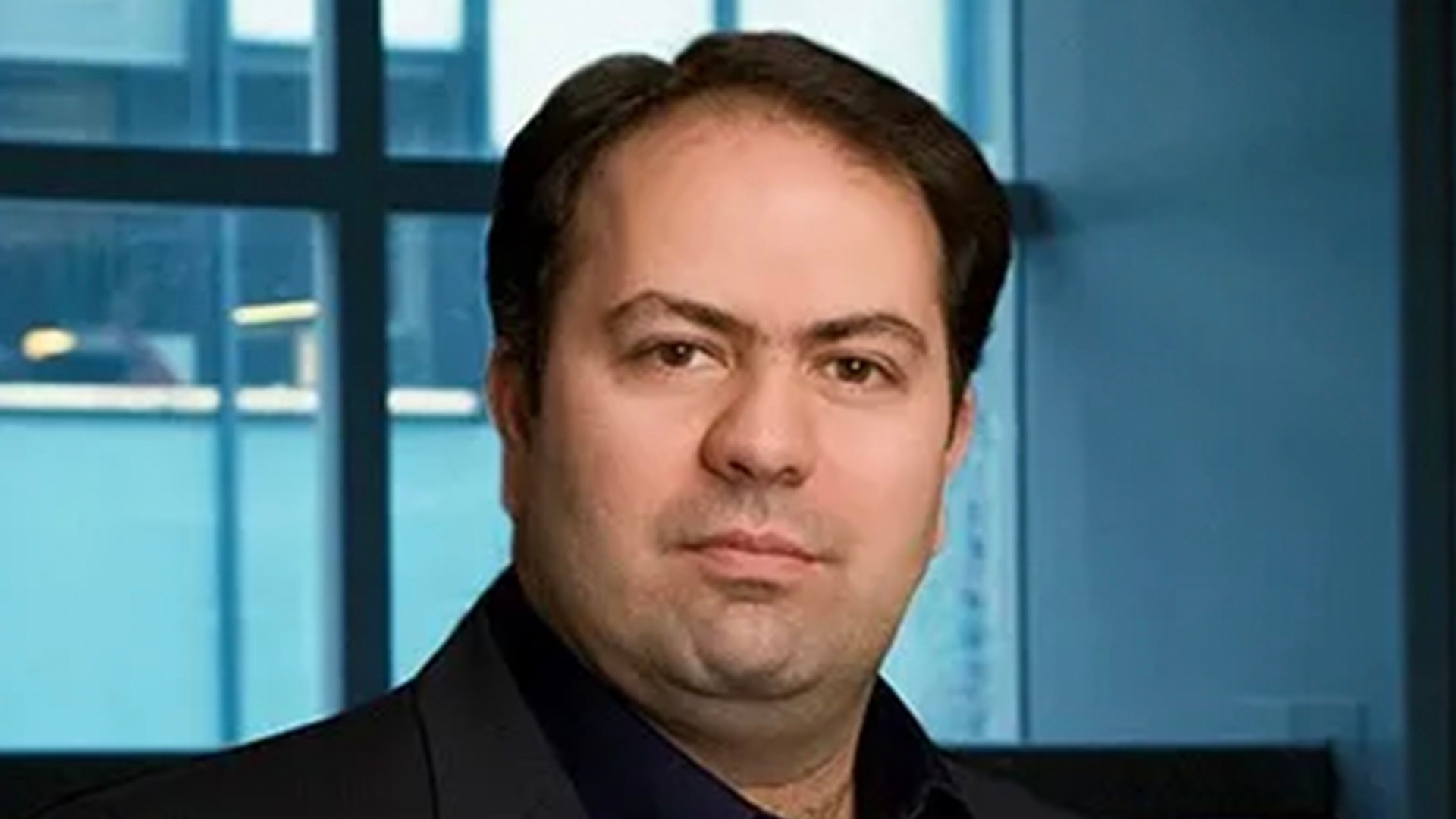
The camera in your smartphone may one day do more than just snap pictures and record videos. A new photonics technology developed by this year’s Bell Labs Prize winner Firooz Aflatouni could turn the smartphone into the ultimate image-recognition device, capable of instantly identifying anything or any context before you ever press the “take picture” button.
While image recognition is by no means a new technology, it’s not something you would typically find embedded in a handheld device. Today image and video interpretation are done by AI/ML in the cloud, which can efficiently and quickly process massive volumes of image data. What’s more, those images must be converted from analog photons into digital bits, which then must be transferred through a suitably high-capacity connection to the image-recognition platform in the cloud. Aflatouni, an associate professor in the University of Pennsylvania’s Department of Electrical and Systems Engineering, has devised a system that eliminates the need for any of those middle steps, bringing image-recognition techniques directly to the source of the image.
Working with University of Pennsylvania postdoctoral researcher Farshid Ashtiani, Aflatouni has demonstrated an integrated photonic-mmWave deep neural network for image, video and 3D object classification. The architecture directly processes raw optical data, obviating the need for any optical-electrical conversion or data transfer.
“Our system performs computation as the light propagates through the system at almost the speed of light and is significantly faster than today’s digital computational platforms,” Aflatouni said.
These image processing capabilities don’t require the might of a large digital processing chip and memory to perform optimally. The system is compact, extremely energy efficient and low cost, which means it can be easily localized in the camera itself. That opens up a tremendous range of new applications for image-recognition technologies in the future. Not only could Aflatouni’s technology turn your camera phone into a sophisticated interpreter of whatever you point it at, but it also could enable smart security cameras that could instantly spot threats and self-driving cars with heightened awareness and deeper understanding of their immediate environs. It could also help protect privacy by operating at lower resolution than GPU-based digital processors, which would only be invoked when higher-order analysis was merited or permitted.
The panel of experts and industry leaders judging this year’s Bell Labs Prize certainly found Aflatouni’s technology compelling, awarding him first prize and $100,000 at a virtual ceremony on Dec. 3. The Bell Labs Prize is an annual competition that seeks to identify disruptive innovations that will define the next industrial revolution. This year Bell Labs received entries from 208 academics in 26 countries. The winners not only receive cash awards of $100,000 (first place), $50,000 (second place) and $25,000 (third place), but they also get the opportunity to continue collaborating with Bell Labs researchers and mentors to further develop their innovations.
“True to the prize’s heritage, we received some of the most innovative proposals we have ever seen to solve critical problems that are confronting humanity,” said Marcus Weldon, Nokia Corporate CTO and President of Bell Labs. “This year’s winner certainly met the criteria by innovating image and video recognition by leveraging deep neural network chips to perform computation as light propagates through the system. By working directly on the light signal, the system is able to perform at nearly the speed of light, making it orders of magnitude faster than the current state-of-the-art in image processing.”
The second-place award in the Bell Labs Prize went to Princeton University computer science professor Sanjeev Arora and his teammates Yangsibo Huang (Ph.D. student at Princeton University), Kai Li (professor at the Department of Computer Science at Princeton University) and Zhao Song (postdoc researcher at Princeton University). Their proposal “How to allow deep learning on your data without revealing your data” solves a significant problem with the lack of privacy in machine learning tools. Their InstaHide solution is a universal method for encrypting training images that is efficient to apply yet has only minor effects on model accuracy. This innovative machine learning technology allows users to share their data to fully leverage the benefits of these models without sacrificing their privacy.
The third-place prize went to Georgia Institute of Technology Ph.D. student Cheng Qi and his teammates Francesco Amato (post-doctoral researcher at Tor Vergata University in Rome, Italy) and Gregory Durgin (professor at Georgia Institute of Technology) for their proposal “Hyper RFID: A Revolution for The Future of RFID”. Their innovative Hyper RFIDs are based on a new type of quantum tunneling diode-based radio positioning system that provides highly accurate wireless positioning with an extended RFID coverage range of meters today, to more than a kilometer in the future. Furthermore, the concept allows for low-cost tags with an extremely long battery life of up to ten years. These revolutionary capabilities for RFID tags add entirely new dimensions for applications like people and asset tracking or drop-and-forget waypoints for the navigation of autonomous drones and vehicles.
For more on each of the awardees’ innovations and their potential future impacts, see the video below: