Giving robots the gift of sight
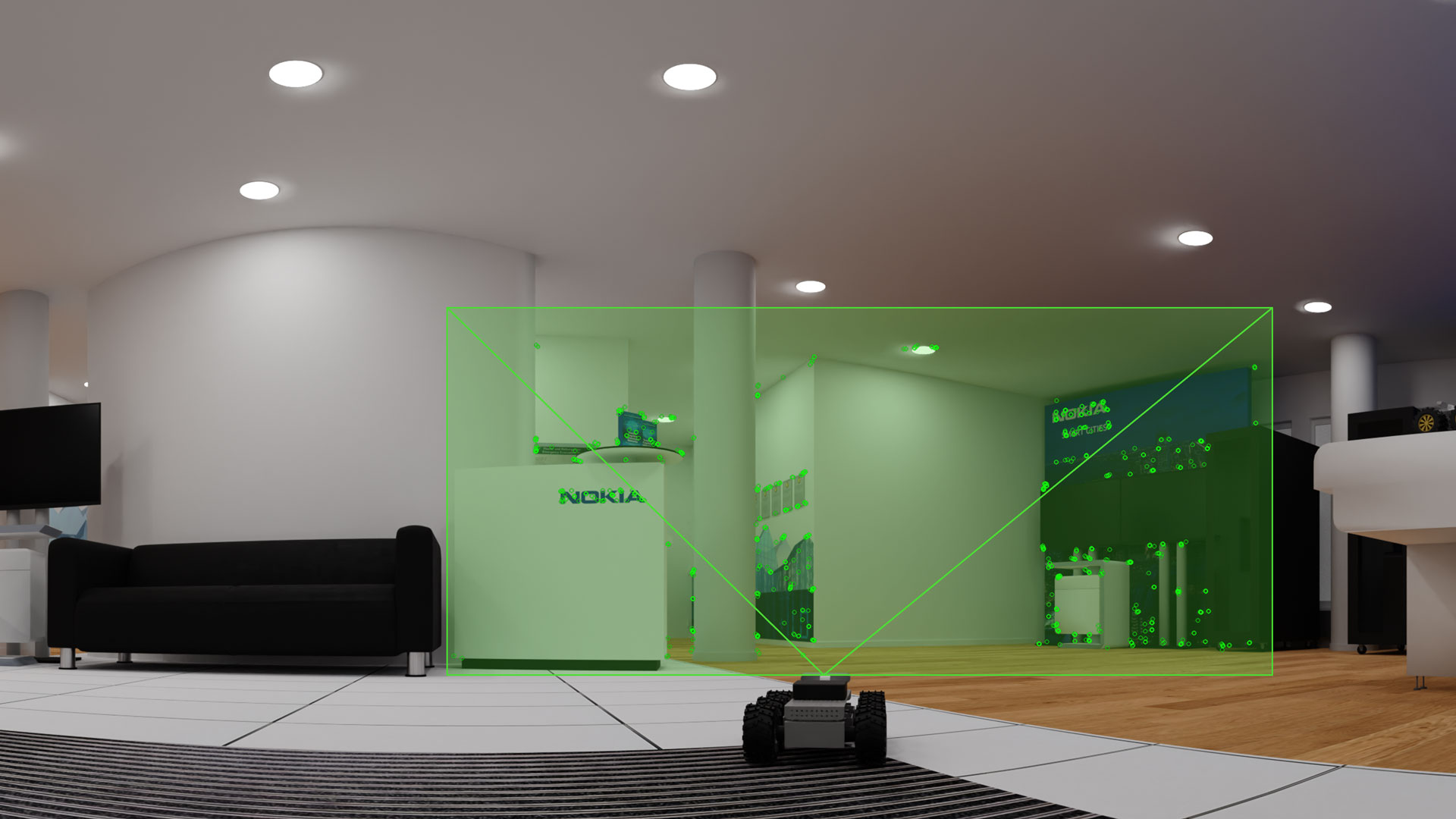
In a lab in Munich, researchers are training robots to sense their environments in ways they never have before. Nokia and Technical University of Munich (TUM) are equipping autonomous mobile robots (AMRs) with cameras to “see” their surroundings and infer their locations the same way humans would. This technology will give these robots a new degree of agility, allowing them to perform intricate parking maneuvers and manipulate items with precision. But most significantly, this robotic vision will give AMRs an awareness of their locations – this has broad implications.
Using AI/ML and edge computing, these robots are creating a detailed virtual map of their environments in real-time – a digital twin. By comparing the images they see in their cameras to that digital twin, the AMRs can determine their precise locations. This leads to a semantic understanding of the robots’ environments, capable of distinguishing specific objects like tables, chairs and people and rendering them as detailed digital representations. These digital twins then could be accessed by numerous other applications.
This TUM-Nokia collaboration is part of Nokia Bell Labs’ Distinguished Academic Partnership program, which marries some of the brightest minds in academia with the industry expertise of Nokia researchers. This research also fits squarely into Nokia’s Tech Vision 2030. In the coming years, digital-physical fusion will be one of the major drivers for advanced networking. The Munich research could provide a path to building the digital twins that will be essential for interlinking the digital and physical worlds.
Furthermore, robotic vision could complement new network positioning capabilities being developed for 5G-Advanced. This could give AMRs and other robots access to both radio-based and semantic-awareness positioning. Together, they could be used to solve a perplexing problem in the field of robotics: how to accurately determine location indoors.
The places satellites can’t reach
Current positioning technologies like GPS and radio-based positioning either don’t work indoors or don’t provide the precision necessary to determine an exact location in a busy, crowded location. But think about how humans determine their location. When you wake up in your bed in the morning after a deep sleep, you instantly know you are in your bedroom as you recognize the walls, the décor and even the blinking light of your alarm clock. By equipping robots with cameras, they could recognize the features of their surroundings much like humans would, said Rastin Pries, research project manager with Nokia Standards.
“We are detecting vertices in the images from those cameras, and in a single image we can detect roughly 1000 of them,” Pries said. “Those vertices are what you might call features, and they help us get to know the environment. By comparing those features with existing maps, the robot can determine its location within a building. In the Munich lab, we have achieved an accuracy of less than 5 centimeters, which is much better than radio-based positioning systems.”
Mounting a camera on a robot is not a new idea by any means. The problem Nokia and TUM are solving is a data problem. When you have hundreds of robots in the same factory, all seeing their environments, the challenge is to process that image data in the most efficient possible way. To create an optimal system, processing needs to be split between the network and the robots operating within it, said Sebastian Eger, a TUM PhD candidate researching robotic vision.
“How do we offload these processing tasks to the edge cloud, and what tasks can we offload? Can we run some tasks on both sides? How do we merge data contributed by multiple robots into a single map? Which data do we need to keep, and which data can we neglect? How do we make that data available to the other agents in real time? These are our areas of research interest,” Eger said. “Ultimately we are focused on creating a collaborative system where we have multiple robots that can all communicate with each other over the network.”
For instance, in a large factory with multiple levels, individual robots don’t need the entire map of the building. As they move between rooms and floors, the network uploads the appropriate map to each robot, which they then compare against their camera data to determine their precise locations in real-time. As those robots encounter obstacles, people or other robots, the calculations become more complex. The robots need to process what they see quickly to avoid collisions or accidents, but at the same time they need to share their data with the edge cloud so other robots can be rerouted or the source of a traffic jam can be isolated. TUM and Nokia are essentially determining the right mix of cloud, local-processing and radio resources necessary to tackle the countless possible scenarios of this nature.
Fusing the physical and digital worlds
It would be a mistake, though, to assume robotic vision is merely a positioning technology. These robots are developing a semantic understanding of their environments, which in turn, constructs a sophisticated digital twin of the world around them. While the robots are accessing map data and resources from the cloud, they are also sharing their image data with the cloud, helping create the very maps they are accessing. The maps they are building aren’t mere floorplans. They are three-dimensional constructs of the spaces these robots collectively occupy, populated with objects, machines goods and even people. As conditions change – people move between rooms, inventory is delivered, a row of boxes topples over – they are immediately reflected in the digital twin, allowing every robot and machine linked into that twin to coordinate their activities.
Such a digital twin has potential far beyond robot orchestration. In an industrial setting, that digital twin could be combined with data from other sensors to create a real-time representation of an entire factory’s operations. In a public setting, cameras in autonomous cars could collectively generate real-time maps of highway conditions down to speed, position and trajectory of every vehicle on the road. We can take advantage of those digital twins in myriad ways beyond their immediate applications. Extended reality (XR) services, for instance, require not just positioning detail but also a deep level of environmental semantic understanding if users wish to virtually interact with their physical environments.
Combined with 5G-Advanced positioning and eventually 6G’s network-as-a-sensor capabilities, robotic vision may very well become a critical component in the broader sensing ecosystem that will digitally transcribe the world around us. We won’t just be teaching robots to see, but also to act as guides as we bridge the gap between digital and physical.
Images from top to bottom: 1) AMR using cameras to sense its location be detecting vertices in the environment, 2) Detailed look at AMR with camera sensors, 3) A dense-point-cloud rendering of a building interior generated by AMR camera data