How social media and cutting-edge AI will help us tackle health crises
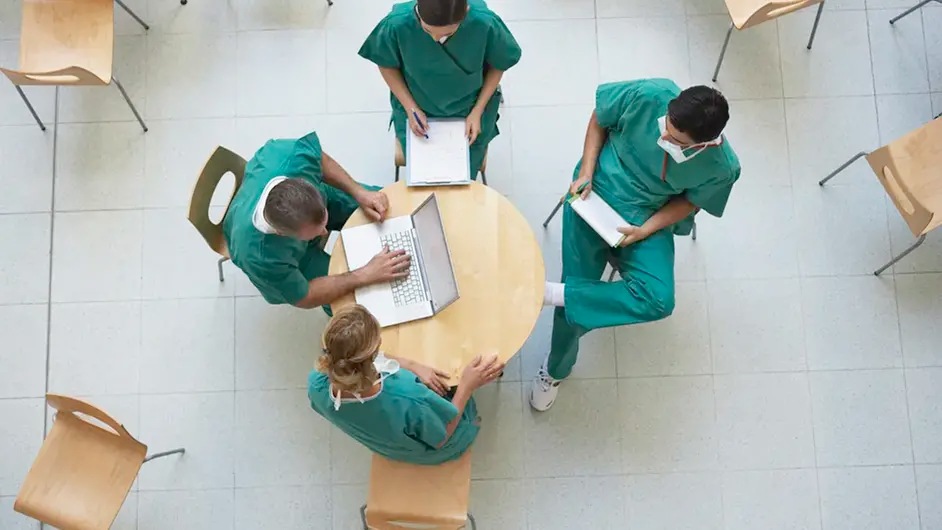
AI can now analyze user-generated internet data to inform public health policy during the pandemic. That’s one of the many use cases for a new AI tool developed by Nokia Bell Labs that can monitor social media like Twitter, Facebook, Reddit and even Glassdoor to mine a wealth of anonymized health data. That data in turn could he used by public health agencies, the healthcare industry and individual companies to solve some of the most compelling problems they face today, from identifying side effects of drugs to tracking the mental health of employees to orchestrating responses to major health crises like COVID-19.
The Social Dynamics group at Nokia Bell Labs in Cambridge, UK, has developed MedDL, a deep learning method that mines massive amounts of text to reliably extract mentions of medical symptoms, diseases and drugs. The method can discover health mentions from any type of text, but social media presents a particularly inviting target due to its omnipresence, even if such data presents a variety of challenges. Social media data is large-scale (spanning the entire world), noisy (full of jargon), and unstructured (free-form text), making it difficult for most text-parsing engines to decipher.
To overcome those challenges MedDL uses state-of-the-art natural language-processing models called recurrent neural networks, which we train to recognize health mentions. To this end, we produced a new training set containing more than 1900 social media posts manually annotated with the health mentions they contained (the dataset is freely downloadable here). Since the very same health issue might be expressed in a variety of ways, MedDL also uses a technique called contextual embeddings to capture the context around each word. For instance, while some people would use the medical term alopecia in a casual tweet, many more would use phrases like “hair loss,” “hair thinning,” “balding” or “hair falling out.” MedDL is able to capture all of those instances by considering their contexts and, as such, group them under the same category.
When applied to a user’s post, for example, MedDL was able to properly capture and quantify mentions of symptoms such as those in bold:
“Hi all, idk about everyone else but my sleep has been super disrupted during this whole quarantine thing. I've been finding it really difficult to fall asleep at night and I often wake up early morning from pretty vivid dreams. [...] I do have some anxiety, but I don't take medications for it because I want to find more natural ways to help it.”
Similarly, for another user, MedDL detected:
“I am having such a hard time right now. I have had my anxiety under control for many years, but this pandemic has really brought it to the surface again. Today has been the worst. I’m shaking right now.”
While those individual words would be meaningless to a tool parsing medical terminology, MedDL is able to extract specific symptoms, issues or states of mind through context, effectively turning a jumbled stream of adjectives, verbs and nouns into a far-reaching health monitoring engine. MedDL makes it possible to monitor community mental and physical wellbeing in ways that were previously impossible to monitor.
MedDL isn’t intended to target individuals’ health issues, but rather use social media to uncover larger trends that could impact broad groups of people, say a patient pool prescribed a particular drug, a company undergoing rapid change or even an entire country dealing with a health crisis. Ultimately, however, individuals will benefit from anonymized health data from that MedDL collects. Let’s look at three specific examples:
Response to pandemics
During the COVID-19 pandemic, many people shared their health conditions and concerns on social media, which has created a unique opportunity to monitor population health at an unprecedented scale. Yet, it is extremely difficult to extract meaningful information from this vast ocean of noisy data. But by applying MedDL on geo-located tweets in the US during the first three months of the pandemic, we were able to mine meaningful data on the psychological toll the crisis exerted on the population.
In the figure above, we tracked most-mentioned medical conditions on Twitter and estimated their prevalence over time. We found that the pandemic was linked to the emergence of post-traumatic stress disorder-related symptoms (PTSD), including disturbed sleep, feelings of isolation, irritability, guilt, fight-or-flight responses, disturbing thoughts and mental distress. Based on these assessments, a public health agency could intervene in areas in need by offering them targeted relief, for instance putting financial resources into local hospitals or establishing triage locations in pandemic hotspots.
Adverse reactions to drugs
To see how MedDL helps monitor adverse reactions to drugs, consider Amanda, a healthy woman of 60. She caught the flu during winter. After a week of medications, she did not recover and instead experienced a sharp decline in mental function, including unexplainable repetitive movements of her arm. Her doctors could not explain the origin of the symptoms, yet, her son found that similar conditions were reported by Reddit users who were taking both antidepressants and pain medications containing serotonin. His mother was indeed suffering from serotonin syndrome, and she returned to her normal condition after quitting the pills.
Currently, information on adverse effects of drugs for individuals and populations is managed by the World Health Organization (WHO) based on data submitted from pharmaceutical companies, from spontaneous reports from doctors and, on rarer occasions, from patients directly. MedDL can automatically discover adverse reactions from online patients' stories by studying combinations of pharmaceuticals and symptoms often mentioned together. The figure above provides a snapshot of how MedDL correlated specific effects with different drugs and supplements. Pharmaceutical regulators and drug companies could use these correlations to trigger red flags on unforeseen side effects for specific drugs, helping patients in situations like Amanda’s before a prescription is ever issued.
Psychological responses at work
By analyzing anonymous reviews of corporations posted on Glassdoor, we were able to identify mental wellbeing symptoms expressed by employees. The figure below shows how those issues manifested among employees in the S&P 500: employees mainly complained about stress, burnout and anxiety but also about some company-specific issues such as cabin fever or work-related injury.
We foresee MedDL applied by companies looking to take stock of overall mental wellbeing in the workplace so they can identify potential areas of concern. Consider Sofia, a manager at a medium-sized international company overseeing three large teams, each in a different location. Their productivity has been down since the beginning of the pandemic, especially on one team. She wants to understand if the reasons for that decline are psychological and perhaps related to the physical effects of working from home. Sofia could apply MedDL on aggregate internal communication to assess the well-being of her teams while preserving their privacy. If her analysis revealed differences in the levels of expressed stress, anxiety or isolation, she could adopt new policies for her teams to help alleviate those problems.
There are many other ways MedDL could be used as a health monitoring tool, and we feel it could help pave the way for a new field called “infodemiology,” the application of user-generated data toward public health policy. In fact, the Social Dynamics group is investigating numerous ways that social media and user-generated data can be applied to solve many of the world’s most compelling problems. The answers to those problems are being generated in places like Twitter, Reddit, Glassdoor, and internal corporate communications. We just need to create the right tools to find them.