One step closer to making the dream of autonomous alarm correlation come true
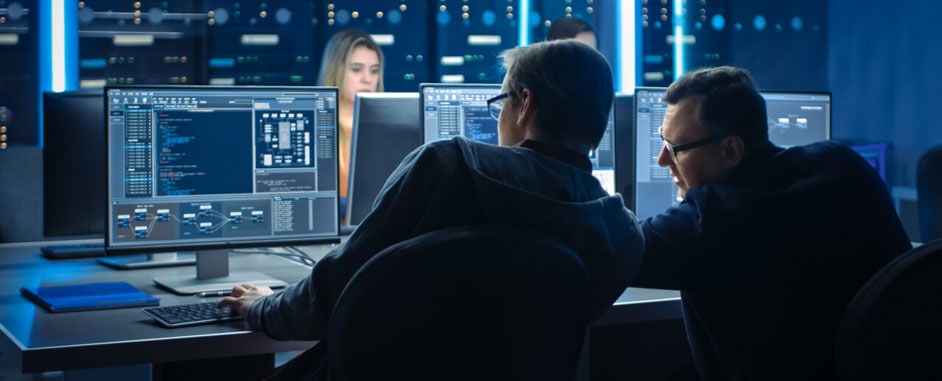
Communications service providers (CSPs) know all too well that increased network complexity translates directly into more complex network management. Part of that complexity comes from the sheer volume of data to be handled, especially related to fault management and alarm processing. Many CSPs would love to get out of the alarm-handling business entirely — not because they don’t care about network-affecting issues but just to avoid the time-consuming tedium of manually managing thousands of daily alarms.
Their wish is closer than ever to coming true. At Nokia, we’re working in partnership with our customers to develop highly intelligent and, eventually, fully autonomous network alarm correlation solutions. “Autonomous” goes a step beyond mere automation: it’s totally hands-off from start to finish, freeing CSPs to focus on other aspects of their network operations.
Escaping “alarm overwhelm”
Automation is becoming critically important because of the complexity that 5G is bringing to networks around the world. Network changes happen more frequently, creating huge volumes and more variations in the kinds of network events to be managed. That leads to increasingly complex rule types that have to be defined and implemented to process incoming alarms — far too complex to set up manually. All the time and effort required to do so is taking away from the value-added tasks CSPs would rather be doing, including enhancing their networks and service offerings.
As an example, today’s cloud-based networks have many interconnected layers of hardware-based infrastructure and virtualized network functions (VNFs). A fault in any one layer can trigger multiple simultaneous alarms across multiple layers. To process those alarms manually, teams need to determine if the VNFs caused the issue, if the virtualization layer is configured correctly, if the hardware is faulty, and so on — for every single alarm received.
Clearly, that makes it hard to quickly and accurately determine which alarms need immediate attention and which can be safely ignored. It also masks correlations that might exist between multiple alarms caused by a single root incident. Beyond the wasted effort in fault handling and isolation, manual processes can also lead to incorrect diagnoses and trouble tickets being sent to the wrong field teams, which compromises efficiency and network quality even further.
This quickly becomes unacceptable at high volumes. Engineers at Vodafone Germany, for instance, were being bombarded with anywhere from 10,000 to 300,000 network alarms a day as the company’s network got more complex. Our pilot project with them to bring some relief ultimately led to the development of our intelligent alarm correlation solution.
Intelligent alarm correlation with machine learning
Our goal with Vodafone Germany was to tailor a solution that would let its engineers focus on only the most critical alarms, reducing their workload so they could attend to more value-added tasks. Our approach was to use machine learning to monitor and triage alarms, identify patterns and common root causes of multiple alarms, and quickly perform minor fixes to maintain network quality. Thanks to the power of big data and affordable computing resources, what we call “network alarm pattern discovery” can augment human intelligence and help bring real value to day-to-day network operations.
Here’s how it works. No matter how many alarms are being pumped into the network management system at any given time, our solution breaks the complex data pile down into discernible patterns so the alarms can be systematically divided and conquered. Sophisticated analytics use machine learning to assess incoming alarms in light of both historical and real-time data, building the solution’s accuracy at identifying patterns over time. Those patterns are then translated into recommended responses that engineers can accept or modify as needed.
Intelligent, repeatable rule creation for alarm processing further supports automation and, eventually, fully autonomous operations. With more data, the rules themselves become more accurate so greater numbers of unwanted alarms are suppressed or consolidated into single incidents, minimizing the volume of network traffic sent to higher OSS/umbrella layers or to service operations where trouble tickets are generated. And with automated ingestion and interpretation of alarms minimizing the number of rules that have to be checked manually (and regularly), CSPs no longer need domain know-how or deep technical expertise in this area.
Just getting started
In our pilot with Vodafone Germany, we found our solution could help CSPs achieve 90% pattern recognition accuracy when analyzing alarms — and reduce the proportion of alarms requiring action by up to 70%. Big picture, that means engineers can work more efficiently. Troubleshooting is simpler, root causes are isolated faster and entire incidents are solved at their source, without any need to address multiple alarms individually.
Our network alarm pattern discovery solution is now available as part of NetAct and will soon be part of Network Operations Master, too.
Going forward, collaboration with CSPs will continue to be key to further enhancing it and similar solutions built around machine learning. Our vision remains to create a solution that processes incoming alarms autonomously, solving network issues without any user intervention at all. Join us as we pioneer the world of network management in the 5G era.
Learn more:
Case study on intelligent alarm correlation with NetAct and Network Operations Master - Vodafone Germany