Stranger things and finding them in Budapest
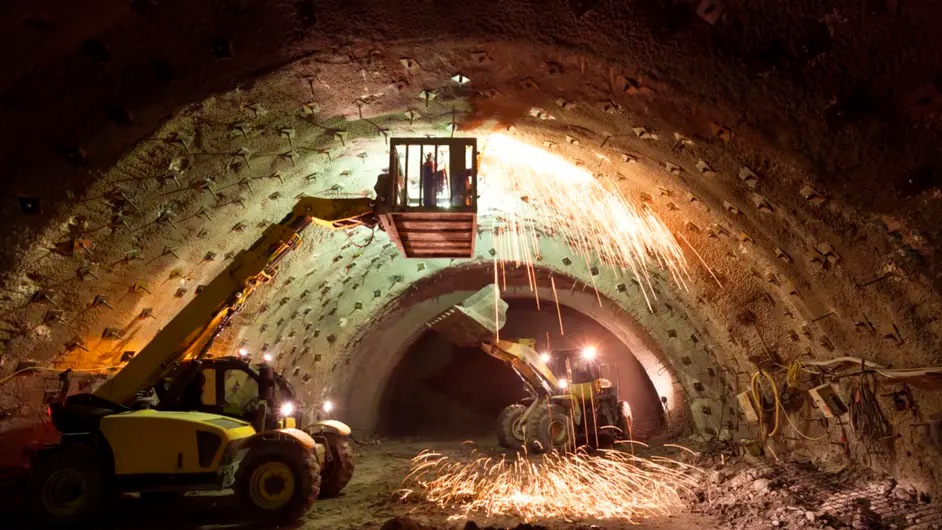
We all know that feeling when we hear a bump in the night or we get a tingling at the back of our neck: the tell-tale signs that something bad is about to happen. Well, Nokia Bell Labs has teamed up with peers at the Budapest University of Technology and Economics (BME) to equip computers to develop the same kind of ability.
As humans, we identify things that bump in the night based on what we expect to happen and what we consider to be normal nighttime sounds. Computers can be programmed to do something similar using algorithms to teach them what is normal and what is strange, known as “outliers” or “anomalies”. Pulling upon thousands (and sometimes millions) of data points, computers can construct a well-defined model of what is normal and then use that model to spot irregularities, which is referred to as anomaly pattern recognition or detection.
While anomaly pattern recognition is nothing new, its use has traditionally been limited to singular tasks. For instance, computers that have been fed hundreds of thousands of faces can be taught to recognize a smile, but they can’t identify the sound of an engine that is beginning to fail. The team of Bell Labs and BME researchers wanted to create a different kind of pattern recognition for detecting anomalies; one that could be applied to many different situations.
Utilizing BME’s expertise in applied mathematics and Nokia Bell Lab’s background in network management, the two have discovered a way for the computer to teach itself, unassisted. The technology is a kind of artificial intelligence that employs machine learning to look for patterns. Unlike competing methods, it uses other detection metrics than Euclidian distance for earlier detection of degradation. It then uses advanced mathematical algorithms to shape the data into multi-dimensional models. In the case of one network, this resulted in a 430+ dimensional space. This ensures that no data is filtered out, which is often true of other methods. The model is constantly being adjusted as more data is entered. Thus, the machine perpetually improves upon itself.
The new technology’s first challenge was aimed at finding network anomalies. In the case of one European mobile network, hundreds of sensors monitored 4,200 nodes of the network using 80 different parameters. The BME/Bell Labs technology then worked through this data to model 13 different kinds of main system states, and it found numerous anomalies that had previously been undetected. The anomaly detection system could also localize the anomalies and pinpoint them in the network so that system engineers at the operator were able to adjust the network to improve overall performance.
The technology has since been successfully adopted by high-tech mining companies to monitor the performance of machinery using data collected from embedded sensors. The solution will also be tested for monitoring the electrical propulsion systems of airplanes. Simply by feeding data representing a phenomenon or range of phenomena into it, the computer can learn to identify, predict and, in some cases, solve weaknesses in machines and networks that, undetected, might compromise performance, efficiency, safety or security.
The beauty of the technology is that because it isn’t domain specific — only good for identifying smiling people, for example — it can be used for finding anomalies anywhere in the network, in the core, edge or RAN. And, as we’ve seen it can also be applied in IoT networks to find anomalies in anything from jet engine performance to drilling machinery. The applications are virtually limitless.
The self-learning anomaly detection project began in April 2017 and was already showing its first results in November. Yet, this fruitful collaboration is decades in the making. The Nokia Bell Labs team in Budapest has been working for more than 20 years with their colleagues at BME. Together they have helped to create multiple products, many of them still in service including Nokia’s VNF Manager. The team continues to develop the anomaly detection technology, and we look forward to seeing the many ways it can be used in future Nokia solutions.