Troubleshooting at F1 speed
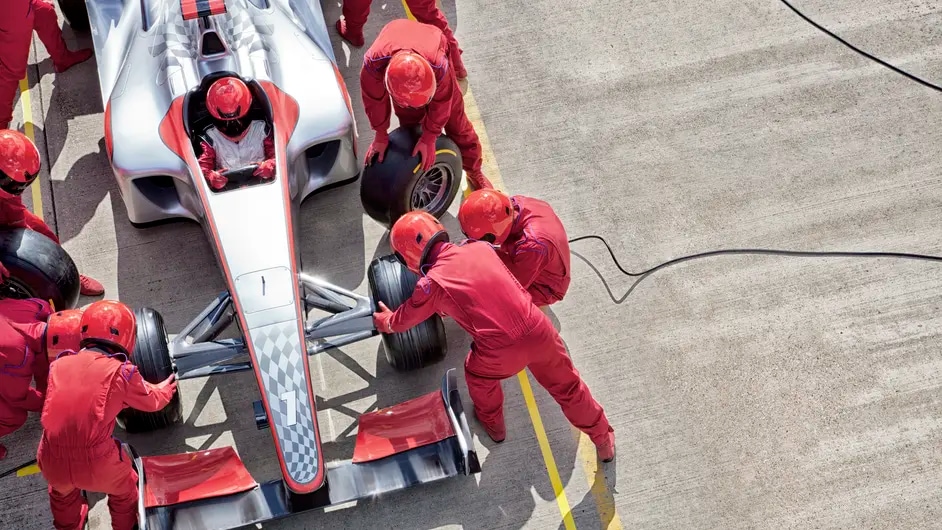
In Formula One (F1) racing, innovation is driven by engineering challenges: how do you design a car’s engine and body to convert power into speed as efficiently as possible? With streamlined processes and tools for developing and testing new features, car designers are now very fast at producing solutions tailored for the unique conditions of the next race to come.
Yet those design improvements have little value without a skilled driver and efficient pit crew to run the race. That’s why the way a driver and crew operate has evolved so significantly over the years, partly by using technology to eliminate human intervention - such as avoiding the need for refuelling at the pit stop - and partly by developing new tools and processes to make pit crews faster and more consistent when conducting adjustments and maintenance. These tools have been critical to augment human abilities to reach, for example, a pit-stop record of 1.82 seconds. A similar augmentation can be done for the crews that maintain telco networks.
Augmenting the “pit crew” of the network
Just like F1 racing teams, network vendors and operators are continuously innovating so they can develop and deploy network functions faster than ever before, adopting modern “DelOps” practices that merge delivery with operations (the telco version of “DevOps” in the webscale world).
This shift toward ever-faster lifecycles is creating significant challenges for network engineers to maintain and troubleshoot carrier-grade software. Although tooling is available for automating deployment, troubleshooting network problems still requires a good amount of manual intervention, causing delays in solving critical issues that ultimately lead to reduced customer satisfaction levels as well as inflated support costs.
As in F1 racing, intelligent new tools will be critical to augmenting network engineers’ speed and operational insights. These tools need to understand the state of customer networks and their operational context to create meaningful event streams that identify operational issues from customer ticket information, narrow down the level of support team required and trigger resolution mechanisms fast.
Faster pit stops with Nokia Cognitive Traffic Analyzer
To augment the capabilities of network engineers, Bell Labs Research and the Nokia Core Services and Care team have developed the Cognitive Traffic Analyzer: a tool built using a new machine learning (ML)-based technology to greatly accelerate network troubleshooting activities. It features flexible, innovative data pipelines and assets for faster customer ticket resolution, and it helps maintenance and support teams identify, analyze and close customer issues by recognizing anomalies against reference data.
The tool automates the complex customer-specific call-troubleshooting workflow, which has traditionally required time-consuming processing and packet trace filtering to manually detect protocol anomalies in network components for different call-flow scenarios.
Offered as a web-based service to Care engineers, the Cognitive Traffic Analyzer fully integrates automated processes for ingesting and normalizing huge volumes of data about the health and performance of network functions and uses ML algorithms to remove defects and accelerate troubleshooting. It takes call-flow traces as input and automatically identifies the participating network elements, then detects and presents anomalies in the call flow to help engineers pinpoint and resolve network issues quickly.
Figure 1: Cognitive Traffic Analyzer
The customer tickets analyzed by the tool are not just resolved — they automatically feed back into the solution’s ML algorithms, helping it learn more complex interactions and anomaly patterns across deployments that are not easy to detect by the human eye. The call-flow traces used by the Cognitive Traffic Analyzer are easily available across customer-specific deployments, resulting in a universal solution for any kind of needs related to call-flow analysis.
Resolving tickets at double the speed
We expect the Cognitive Traffic Analyzer to cut the time it takes to troubleshoot network issues in half by automating routine maintenance as well as more complex scenarios. That speed is likely to improve over time with usage as more and more scenarios are learned by the machine. That means the time savings will tend to improve over the first couple of years of usage before stabilizing at an optimum value.
Figure 2: Cutting ticket resolution time
This technology will augment network engineers’ ability to predict and quickly react to any errors in the network, leading to improved customer satisfaction and a better overall experience for end users. Customers will enjoy the insights and benefits of this tool through Nokia’s Care services.
It represents another step forward in Nokia Core Services and Care’s DelOps vision, allowing for the necessary automation of the testing and support phases so that network maintenance and troubleshooting can happen at “F1 speed”.