Using GenAI to build a language model for network automation
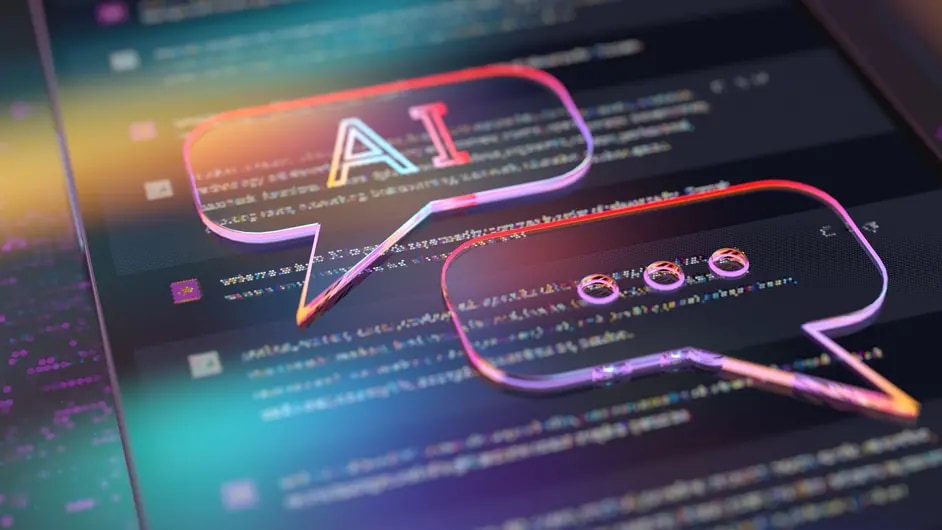
I wonder what took me so long to blog about AI and networks. As chief network officers think, talk, and act on networks – could I think and talk with them in their language? AI is about language and connecting their language with their networks may uncover endless opportunities for new customer interaction and creative thinking. Having spent several years investigating how AI can help our customers, I am thinking disruptively across all aspects of the life of a network. It is not just about routers and line rates and other cool technologies. It’s more about how we talk about these technologies.
Why AI requires us to think differently
In essence, AI is all about semantics and data, which together bring new ways to express disruptive insights and sharp actions by humans and machines.
Semantics is about picking up everything that was said between stakeholders, recording it and identifying how it all fits together. A lot of research has been conducted around the concept of semantic spaces. This is the space, or set of attributes, in which the meaning of a concept is expressed. The textbook example is the word bridge, which in the telecommunication industry means an intelligent device that receives, processes and transmits data from one network to another. A ship’s captain may have difficulty relating to this type of bridge while overlooking the wild sea from the bridge of his ship.
As we do our everyday jobs as network designers, business-to-business sales engineers or chief network officers, we wander across adjacent and overlapping semantic spaces. For example, the meeting with operations brings its own tone, while the afternoon meeting with a not-so-happy customer brings other associations between words.
Note that the language used will vary greatly—from the body language of the customer, to the code of the developer that unambiguously expresses the intent through an algorithm, the alarm that a network management system reports, and the graph that the chief network officer uses to show the board of directors how the quality of the company’s transport network relates to its net promoter score. Together, these semantic spaces roll up into broader spaces that may be connected, such as IETF standardization, or not connected, such as vendors’ cost data or service providers’ competitive offers.
Data starts with logging what can be measured, converting it to specific formats and structures, analyzing it, and drawing conclusions from patterns. Advanced analytics allow network planners and operations teams to anticipate outages, link congestion and other events that would impact the customer experience. Used across organizations and stakeholders, Extract, Transform, Load (ETL) is a data integration process that extracts data from multiple sources, transforms it and loads it into a data warehouse or other unified data repository.
In the era of generative AI (GenAI), the concept of data gets an even broader definition. Everything that can be recorded is data: pictures, words, code, comments, error codes, music, sounds and more. As we design, plan and operate networks, we can use everything that was said, every test that has succeeded or failed, every incident that has happened, every industry standardization document and all the arguments about why one network design was chosen over another. It’s all data.
Leveraging AI towards autonomous networks
Every so often over the last 30 years, I have written a blog post, and all of them have included the word complexity. My streak continues with this post. This decade has brought new dynamics and complexity into networks. A node-centric view of networks is complemented with software and API perspectives, domain segregation (WAN, access, xhaul), interleaved transport and data center networks, evolving technologies driven by new use cases such as ultra-low-latency graphics processing unit as a service (GPUaaS), and more demanding services up to dynamic network as a service (NaaS).
Each step of this evolution is driven by new business cases and the availability of new capabilities. But adding them up into one single service-level agreement (SLA)-defined experience remains the eternal challenge. TMForum frames this challenge as zero X, meaning zero wait, zero touch, zero trouble.
According to TMForum’s vision for Autonomous Networks (IG1229), the path to zero X requires machines in general, and GenAI in particular, to come to the rescue. The data is there—somewhere—that explains the intent of a network service, the SLA it needs to meet, the proof that the SLA has been met, and the potential actions required if the SLA has not been met. This is expressed in various forms of languages.
The semantics point to the meaning, properly brought together through data lakes. Large language models will discover the relationships across meanings, and reason towards potential steps for remediation. It all comes together in a language through which machines and humans can talk to each other, and together identify and execute the right actions. The entire process is human owned but machine-assisted.
NSP has a mandate to speak the CNO’s language
Nokia Network Services Platform (NSP) is the automation platform of choice for well over 1,000 network operators. It has entire end-to-end IP networks under its control, from the customer’s premises, over aggregation and xhaul, over gateways of various kinds and up to peering points. NSP can see all data that comes from the nodes across the entire path of the network service and provides software modules that automate configuration, assurance and closed-loop remediation of network services.
The addition of GenAI capabilities allows NSP to correlate data streams in new ways because it provides reasoning that helps NSP understand what it sees, discover new relations across networks, and identify root causes of events. Through ongoing work to develop an ‘NSP language model’ derived from large language models, we’re bringing a series of semantic spaces together across the whole spectrum of NSP’s capabilities, from product line management to code and test generation, and to network service authoring and validation.
Our vision is that the GenAI-enabled NSP will act as the assistant to our customers’ network designers, planners and operations staff. It will help them extend the ‘NSP language model’ towards a ‘CSP network language model’ that is private to the CSP. This vision culminates with the generation of a specific report that the chief network officer can show to the board to illustrate the quality of the CSP’s transport network in relation to its net promoter score.
Find out how we are integrating GenAI more extensively into NSP for automating network operations: Nokia IP networks and AI