Networking for AI workloads
Build data center fabrics that meet the demands
of current and future AI applications
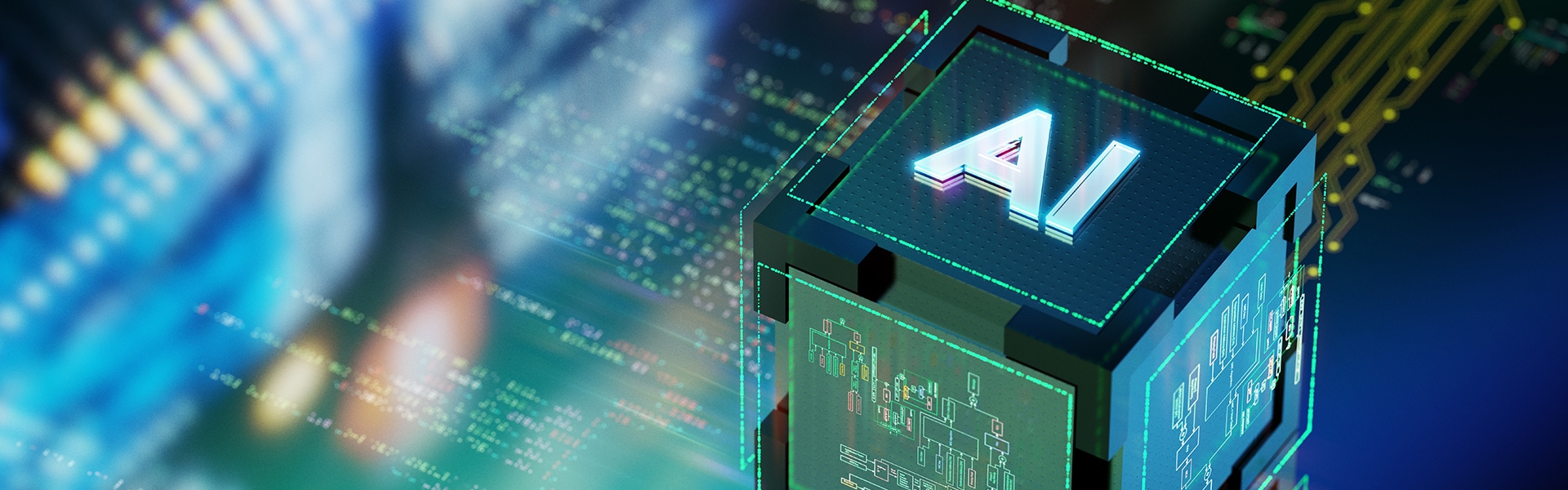
What is networking for AI workloads ?
Artificial intelligence (AI) is changing the way businesses work. Companies of all types are embracing AI applications that promise to increase operational efficiency, transform the user experience and unlock new revenue. Networking for AI workloads helps them realize this promise by implementing data center networks to support compute- and data-intensive AI infrastructures.
What makes AI workloads different?
Most AI workloads are more compute-intensive than workloads created by traditional computing applications. Many also involve the exchange of massive volumes of data. All require fast processing to deliver the experiences users expect.
There are two main types of workloads associated with AI models. AI training involves data collection, model selection, model training and model deployment. AI inference involves deploying the trained model so it can serve users and respond to their input or queries with suitable output. For some applications, this output needs to be generated in real time.
To meet existing and evolving AI needs, compute nodes must be interconnected by high-speed, lossless and low-latency networking. This is essential to reduce JCT, a metric used to measure the time it takes to complete a task, such as training a model or performing an inference operation.
Back-end and front-end networks for AI workloads
Organizations need well-designed data center networks to tackle the challenging demands of AI workloads. These networks must extend seamless, reliable connectivity across the AI infrastructure and deliver the best possible performance for every AI training and inference task.
The back-end network is used to interconnect the high-value graphics processing unit (GPU) resources required for high-computation tasks such as AI training, AI inference or other HPC workloads. It delivers lossless, low-latency and high-performance connectivity for AI training compute and dedicated storage resources.
The front-end network supports connectivity for AI workloads, general-purpose workloads (non-AI compute) and the management of AI workloads. In the context of AI inference, the front-end delivers fast response times and proximity to the end user.
In both cases, it’s essential for organizations to control—and, ideally, reduce—their cost and power use as they deploy networks to meet these new demands.
Ultra Ethernet Consortium (UEC)
More and more organizations are choosing Ethernet technologies to build back-end networks for AI workloads. Ethernet already dominates front-end network designs. The work of the Ultra Ethernet Consortium (UEC) will bring enhancements that make Ethernet the best choice for AI network infrastructures.
UEC is working to deliver an open, interoperable, high-performance, full-communications-stack architecture based on Ethernet to meet the growing network demands of AI and HPC at scale.
UEC members, including Nokia, aim to leverage the ubiquity, performance curve and cost benefits of Ethernet to evolve the legacy RDMA over Converged Ethernet (RoCE) protocol with Ultra Ethernet Transport (UET). This modern transport protocol is designed to enhance network performance to meet the requirements of AI and HPC applications while preserving the advantages of the Ethernet/IP ecosystem.
How can Nokia help you implement networking for AI workloads?
The Nokia Data Center Fabric solution provides the reliability, simplicity and flexibility you need to build and deploy network infrastructures that can meet the requirements of current and future AI workloads.
Modular and fixed-configuration platforms
Our solution includes a comprehensive portfolio of modular and fixed-configuration hardware platforms for implementing high-performance leaf–spine designs. You can use our platforms to build high-capacity, low-latency and lossless back-end networks that can efficiently handle demanding AI training workflows. We also offer platforms in many different form factors to support front-end network designs that will interconnect your AI inference compute, non-AI compute and shared storage resources.
A powerful and proven network operating system
Our data center hardware platforms run on the Nokia SR Linux network operating system (NOS). SR Linux opens the NOS infrastructure with a unique architecture built around model-driven management and modern interfaces. It is designed for reliability and quality, ready for automation at scale, and easy to customize and extend.
SR Linux provides congestion management and traffic prioritization capabilities that let you deliver lossless Ethernet networking. It also supports high-performance AI infrastructures with superior telemetry, manageability, ease of automation and resiliency features.
Fabric management and automation toolkit
Nokia Event-Driven Automation (EDA) is a modern data center network automation platform that combines speed, reliability and simplicity. It makes network automation more trustable and easier to use wherever you need it, from small edge clouds to the largest data centers.
With EDA, you can automate the entire data center network lifecycle from initial design to deployment to daily operations. This allows you to ensure reliable network operations, simplify network management and adapt to evolving demands.
Data center interconnectivity
Some companies may implement their own training infrastructures. Others may prefer to use AI platforms and associated services from large cloud providers, an approach known as GPU as a service (GPUaaS) or public AI.
Inferencing models that need low-latency end-user access are typically run in enterprise edge locations in private AI infrastructures. Batch-mode and global-scale inferencing models are better suited for implementation in public AI frameworks.
We offer a comprehensive solution that makes it easy to interconnect AI infrastructures between data centers and across the WAN. The Nokia Optical Data Center Interconnect (DCI) and Nokia Data Center Gateway (DCGW) solutions let you provide reliable, high-performance interconnection across AI infrastructure domains. They can help you meet evolving distributed AI connectivity requirements so you can serve AI models at the edge of the network, closer to end users.
Learn about networking for AI workloads
Discover our related networking solutions for AI workloads
Solution
Data Center Fabric
Bring new levels of reliability, simplicity and adaptability to your data center switching and cloud infrastructures.
Solution
Data Center Gateway (DCGW)
Learn how the Nokia Data Center Gateway can help you optimize data center connectivity, performance, scalability and reliability.
Solution
Optical DCI
Implement business-critical data center interconnect to support your enterprise private/hybrid cloud.
Related topics
Learn more
Video
Nokia and Lenovo – A partnership driving advancements in data center solutions for the AI era
Video
What is Nokia’s EDA platform?
Video
What pressures do AI workloads place on the data center network?
Latest news
Ready to talk?
Please complete the form below.
The form is loading, please wait...
Thank you. We have received your inquiry. Please continue browsing.