Artificial intelligence explained
19 December 2023
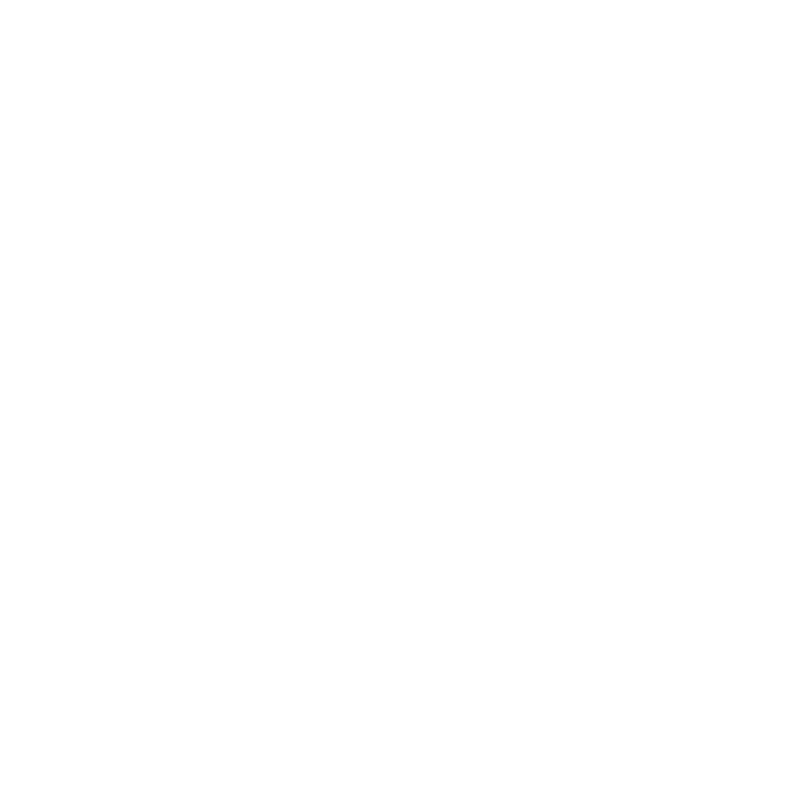
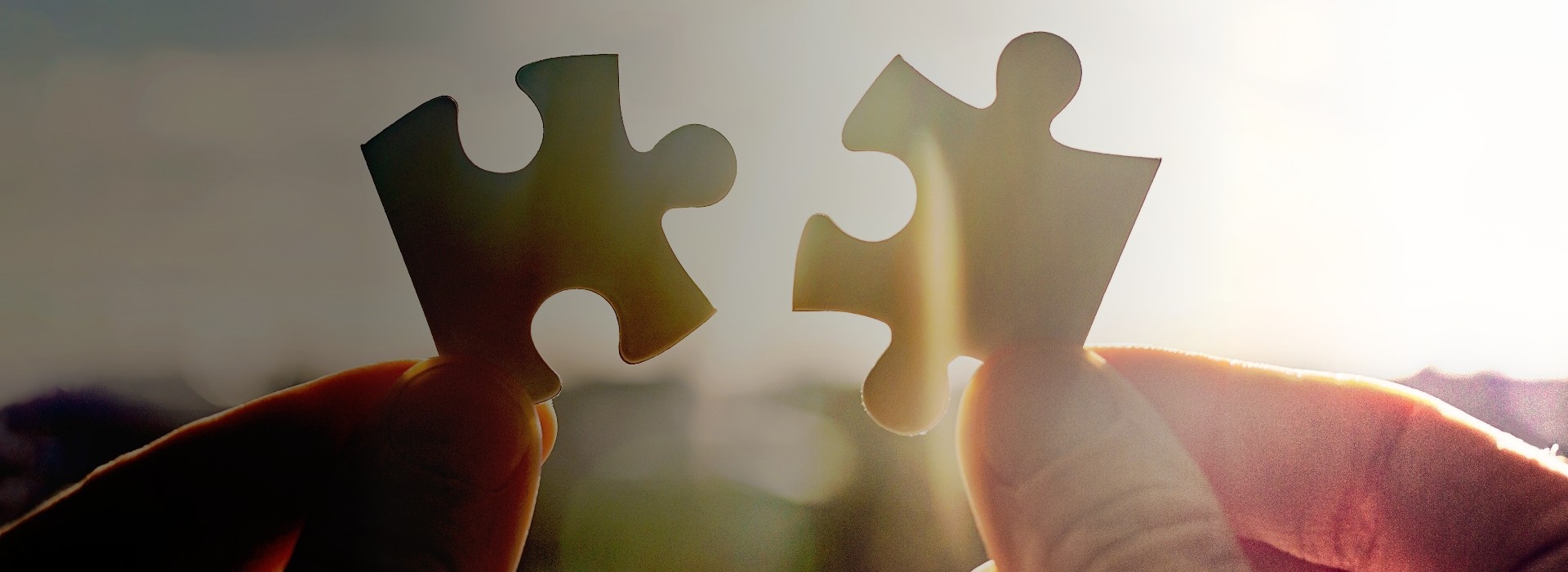
Artificial intelligence or AI is a technology that has been around since the early work of pioneers like Alan Turing and Nokia Bell Labs’ own Claude Shannon. Shannon co-authored the paper that started the formalization of the AI field, and his Logic Gate/Theseus mouse project utilized an early form of AI to learn and subsequently navigate a maze. Practical versions of learning algorithms have been used in networks since the late 1970s, for instance, when they were shown to improve network performance in telephone traffic routing and control.
Today, AI agents are ‘trained’ by analyzing huge amounts of data on the system or process that it is being used to predict or control. The data might simply be raw measurements, digital sound, digital images, video or other inputs. The input data can be unlabeled or may be labeled by human or machine. The goals of the AI agent can be quite diverse and include prediction, perception, language, robotics, and decision-making. AI agents can be trained to drive cars, chat with customers, improve cybersecurity, find information, write reports, diagnose medical conditions, aid in drug discovery, or compete in single player or multi-player games.
Powerful classes of AI algorithms, such as neural networks, require vast amounts of data, making them compute intensive. It is only with the substantial and requisite growth in processing power in the last decade that artificial intelligence/machine learning (AI/ML) has been more widely deployed in networks and in many other fields. Today Nokia uses AI/ML extensively to make networks more reliable, secure and sustainable, while our researchers are actively exploring new responsible uses for the technology.
ChatGPT and generative AI
With the launch of ChatGPT by OpenAI in November 2022, AI went mainstream, and started a very public debate around its use. ChatGPT is a Large Language Model (LLM) powered by the Generative Pre-trained Transformer (GPT) architecture. LLMs are in the category of AI algorithms called “generative AI” that are capable of extraordinary new content creations. In the case of ChatGPT this means primarily text, but similar models also generate audio, code, images, and videos. What grabbed the public’s attention is the uncanny ability of ChatGPT to mimic human language use and respond appropriately and at length to questions on almost any subject. It appears at times to have human-like intelligence.
This has brought into the open concerns around the use of AI. What worries people is the way generative AI can veer into “hallucinatory” states, where it generates disinformation with the same confidence and conviction as it imparts useful, true information. This triggers a long-standing counter-narrative about AI, where upon reaching a generalized intelligence, it is feared that AI might be able to assume agency, pursuing its own ends, not our own.
Despite this uncanny facility to mimic intelligence, however, general intelligence currently remains in the realm of science fiction. Generative AI’s mistakes are usually due to the quality of, and bias in, the data and algorithms which they have been trained on, and the kinds of open-ended questions that users prompt. The ease with which ChatGPT can generate disinformation, nonetheless, reminds us that this amazing technology needs to be developed and used responsibly.
Using AI responsibly
AI can exhibit remarkable accuracy or merely provide a better approximation than a human could manage. Either result can be useful, but a responsible use of AI must account for the margin of error and make allowances for it. Also, is the dataset complete? Sometimes people will collect data on a phenomenon, but because they don’t fully understand it, they will miss data on a crucial variable. This means the AI might generally be accurate, but then wildly inaccurate every once in a while when that missing variable is at play. Finally, as briefly touched on regarding ChatGPT, there is the potential of problems regarding the quality of data that the AI is trained on. It is critical that in training AI, the data is good and consistent. As the old data science adage goes, “garbage in, garbage out”.
Training-data problems like these vary widely depending on the application. The vast majority of industrial use cases for AI carry little risk because the process where the AI is being employed is relatively narrow. These fixed-purpose AIs deal with a limited and known set of data. For instance, in a communications network, telemetry data from user devices, network nodes, servers and applications are the only relevant data. In this case, the AI is being used because the amount of data is vast, and the interactions between the different network elements is too complex to model. At the same time, the relevant data are generally understood and accurate.
On the other end of the scale, there are AIs that don’t have a fixed purpose. Generative AI models such as ChatGPT fall into this group. They are trained on large quantities of data where the accuracy and worth of the actual content is wildly variable and the questions posed are open-ended. Because in its data set there are both good and bad answers to any question, depending on how the conversation goes, the chatbot might be directed to filter for the bad answers over the good answers, leading it to create sentences that communicate untruths, fake news, conspiracy theories and worse. These open-ended AI systems can pose risks to human rights, privacy, fairness, robustness, security and safety.
Using AI in networks
The AI/ML applications used in networks fall into the fixed-purpose classification and many have been around for decades. The concept of self-organizing networks (SON), for instance, was introduced with LTE in 3GPP Release 8 in 2009. With SON, AI/ML is used to automate planning, configuration and management of mobile networks. A leader in SON applications, Nokia research on SON is ongoing, with the goal of automatically optimizing and healing networks before customer-affecting issues occur.
AI is also playing a big role in network security as it can analyze large volumes of data in real-time to identify subtle patterns and anomalies that are often missed, improving accuracy and speeding up detection. Nokia’s telco-centric eXtended Detection and Response (XDR) solution NetGuard Cybersecurity Dome uses AI/ML algorithms to analyze and identify potential security threats, enabling real-time detection and response to security incidents.
Nokia also uses AI in communications networks to optimize power consumption of both active and passive network equipment. Nokia AVA Energy Efficiency enables operators to achieve as much as a 30% reduction in power use. And research into further uses of AI in future networks includes the possibility of predicting usage patterns and turning on and off components even at the processor level to optimize energy use.
In the planning for future networks such as 6G, we envision AI/ML will be embedded in end user devices, radios and radio access networks (RANs). Central to our research on these future use cases is the responsible use of AI. This is a concern across the industry and among regulators and standards bodies with whom we work closely, which is why we developed our Responsible AI principles and encourage the industry to adopt them. We believe these principles should be applied the moment any new AI solution is conceived and then enforced throughout its development, implementation and operation stages. Rather than being perceived as a set of rules and guidelines that limit innovation, these six pillars should be seen as an opportunity for creating the collaboration that AI requires.
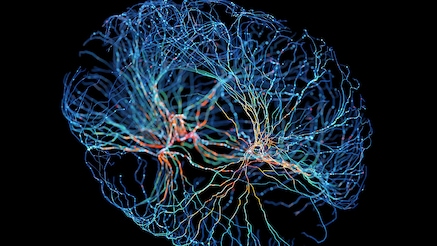
AI networks
Build AI-powered networks that meet the demands of AI applications.
The six principles of Responsible AI
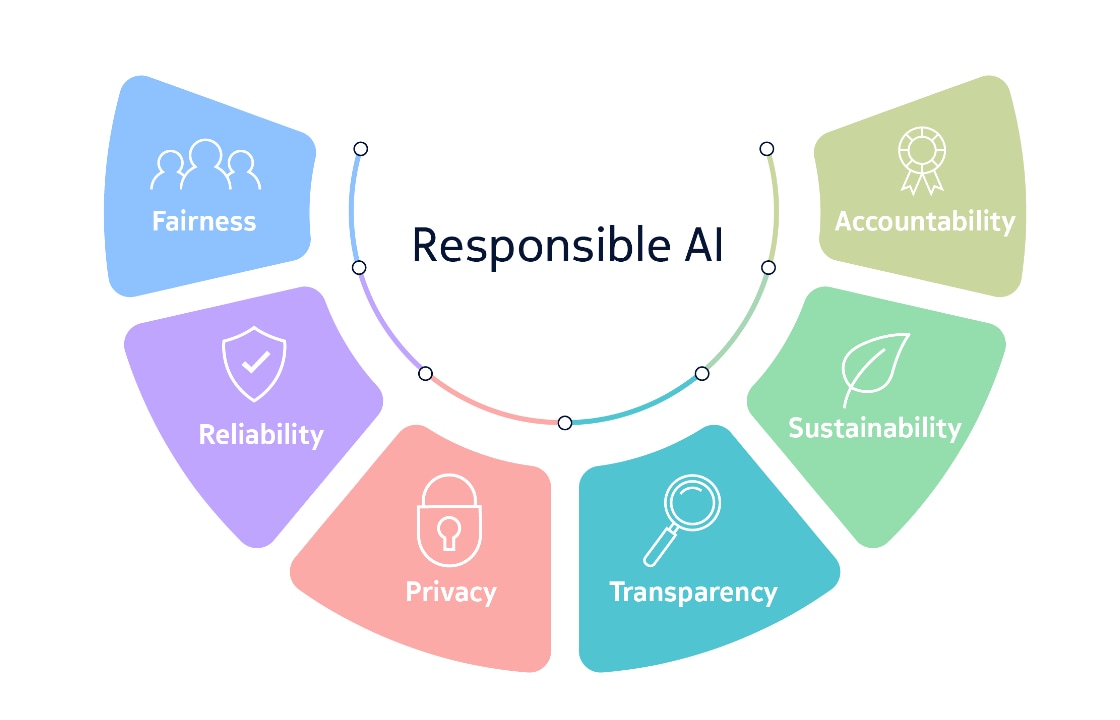
AI will be a critical technology in the future as our world becomes increasingly complex. Many of the technologies that will be key to water management, autonomous transportation, smart cities, clean energy and communications will rely on AI. Narrowly framed, fixed-purpose AI applications have proven their usefulness and safety over many decades. Used responsibly, they will be a critical technology in future networks and will help to make our world more sustainable, safe and secure.
Read more
About Nokia
At Nokia, we create technology that helps the world act together.
As a B2B technology innovation leader, we are pioneering networks that sense, think, and act by leveraging our work across mobile, fixed and cloud networks. In addition, we create value with intellectual property and long-term research, led by the award-winning Nokia Bell Labs.
Service providers, enterprises and partners worldwide trust Nokia to deliver secure, reliable and sustainable networks today – and work with us to create the digital services and applications of the future.
Media inquiries
Nokia Communications, Corporate
Email: Press.Services@nokia.com
Follow us on social media
LinkedIn Twitter Instagram Facebook YouTube