How data is driving the future of PON capacity planning
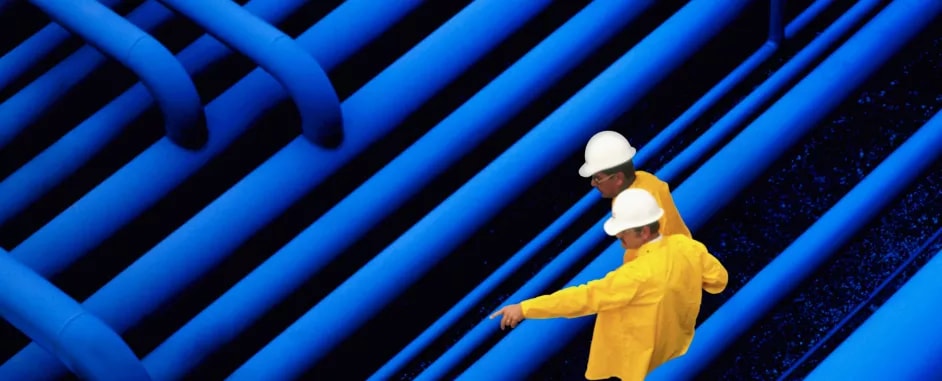
Broadband never stands still. The ever-increasing demand for bandwidth from an ever-increasing user base continues to increase pressure on broadband providers to deliver reliable, high-performance connectivity. A crucial element of ensuring this performance is capacity planning: determining and optimizing network resources, notably bandwidth, needed to meet current and future demands efficiently. However, despite the pace of change in broadband, capacity planning methods have stood still
Established capacity planning practices use a simple formula to assure that, during peak hour, at least one of the PON users provisioned with the highest bandwidth tier can download at full speed.
The formula is: C ≥ (N x T) + (K x PIR).
In this formula, C represents the required PON capacity, N the number of PON users, T the average traffic per user during peak hour, PIR the peak information rate, and K a QoE (Quality of Experience) constant.
The first term of the formula (N x T) allocates sufficient bandwidth for the average peak-hour traffic generated by N users. The second term (K x PIR) is added to cope with traffic fluctuations such that every user has a good QoE. The larger K, the higher the QoE level.
Such a simple formula is understandably limited and imprecise:
-
The formula uses the average peak-hour throughput per subscriber as input. However, residential internet traffic is bursty. The statistical properties of this traffic (variance and autocorrelation) are not taken into consideration. This shortcoming is mitigated by the QoE constant K, but such a mitigation may result in an overly conservative service offering and split ratio.
-
Because traffic fluctuations are not modeled, the formula cannot estimate the risk of congestion.
-
The impact of the PON downstream scheduler (WFQ) and traffic shapers is not considered.
-
Due to game and video downloads (for example, a whole series), long-lasting full-speed downloads occur frequently. Established capacity planning does not account for such heavy usage.
-
New techniques to mitigate the negative impact of long downloads on the congestion risk such as bandwidth sharing optimization (BSO) are not factored in.
Thanks to the arrival of software-defined access networking (SDAN), network controllers can monitor a much larger number of network devices, with AI/ML capabilities processing many more data points – up to 20x more counters, in fact – than legacy network management system (NMS). Data can be collected at high frequency e.g. 5 second intervals, sufficient to enable real-time monitoring, optimization, automation and other programmable actions. This can revolutionize PON capacity planning.
-
High-frequency telemetry can be enabled for thousands of subscribers simultaneously, creating a large database of traffic models capturing all the statistical characteristics of the residential internet traffic.
-
These models can then be used to predict the congestion risk. Moreover, we can focus on speed test success probability, which bridges the gap between congestion risk and the user experience.
-
Predictions can involve simulations that include a comprehensive physical model of the PON downstream scheduler and traffic shapers.
-
Using a large set of training data, we can train a digital twin that predicts speed test success probability for all possible network and service configurations – including PON technology, split ratio, peak-hour throughput per user, offered peak rate, number of heavy users, and BSO status.
Using digital twin technology helps to improve capacity planning and can result in increased PON utilization: up to 33% higher peak rate or double the number of users. And to make it easily accessible to operators, we’ve implemented it in a new Altiplano application called the PON Capacity Planner.
This app provides network design and capacity planning recommendations. It can run what-if scenarios to accurately predict speed test success probability for a current or target configuration. Moreover, the speed test success probability is also predicted for every active PON in the network. The app tracks the evolution of speed test success probability over time and generates an alarm when speed test success is not assured. A top-10 of PONs with the lowest speed-test success probability is provided. All this enables a proactive remediation of a low speed-test success probability, for example, by upgrading a heavy user from GPON to XGS-PON.
The patent-pending PON Capacity Planner application is now available through the Altiplano Application Marketplace. Check it out!