Learn how AI and graph databases transform telecom inventory solutions
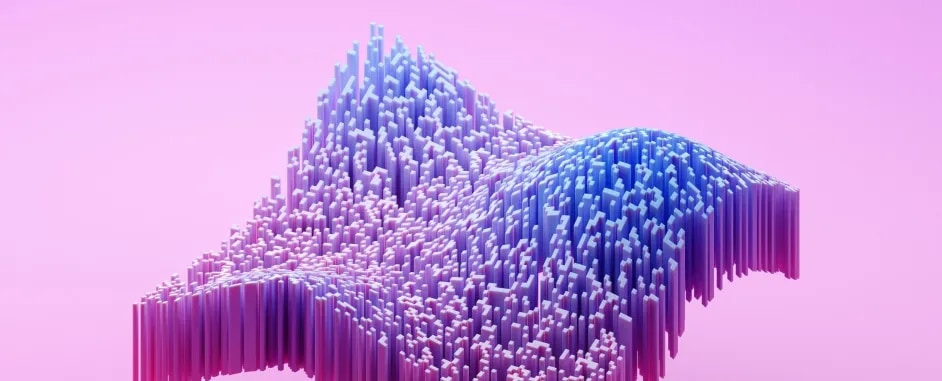
The evolution of data storage and consumption over time is undeniably fascinating. But how did we get to where we are today? What does the future hold? And what does that mean for telecom inventory management?
Evolving from centralized, meticulously organized and structured monolithic solutions, we moved to holding vast amounts of unstructured data in dedicated "lakes" until that proved inadequate as well. Present-day application and service design leverage edge and multi-layer principles:
-
Services are abstracted over various resource and technology layers
-
Services are situated as close to the end-user as possible
-
Disaggregated decision points provide essential inputs at the edge, enabling immediate responses to service performance changes or customer requests
-
Flexible design of distributed architectures that can scale horizontally enables service execution close to the end-user
Why telecom inventory solutions must evolve
The consequence for telecom inventory management solutions? They need to transform from simple data storage systems to data discovery engines, from manually structured data to auto-correlated data and from being static to be near real time.
Next-generation applications often require immediate responses, but this is not possible with centralized decision-making. The 3GPP distributed slicing controller architecture is one example for such an application. But this also applies to applications such as extended reality, energy efficiency, artificial intelligence (AI) / machine learning (ML) and others. Vertical industries are embracing such modern approaches for diverse use cases including transportation, manufacturing, health and more.
Private networks, coupled with applications executing specific tasks on mobile edge devices, are gaining popularity among large enterprises. Despite the proliferation of distributed services, there remains a need for inventory systems to aggregate data at certain points for trend analysis, forecasting, enhanced root cause analysis (RCA), service impact analysis (SIA) and more.
The game changer: Graph databases
To meet these growing data aggregation and storage needs, technology has evolved from traditional relational databases to innovative solutions like graph databases. By prioritizing relationships, they allow direct data linking and enable efficient data retrieval. Graph databases offer techniques for data integration and information sharing, facilitating complex metadata representation and providing rich semantics for natural language processing. Additionally, they are instrumental in constructing knowledge graphs and managing master data.
These advancements are indispensable for addressing complex scenarios as shown in the diagram below. RCA and SIA, for instance, leverage advanced causal discovery techniques to unveil hidden relationships between key performance indicators (KPIs), enabling valuable insights for optimizing network performance and enhancing the effectiveness of automation agents.
Forecasting represents another area where AI uses inventory data, capacity plans, social events and demographic data to predict usage patterns. These predictions can prevent outages or congestion and aid planning efforts by providing data for simulations and what-if scenarios.
What-if scenarios and forecasting also serve as the foundation for network digital twin applications. Closely resembling real networks, network digital twins are useful for operations, planning, optimization and maintenance purposes, like the prediction of the behavioral impacts of changes to network, traffic, service, or environmental conditions.
Conclusion
To enable autonomous networks, telecom inventory solutions need to evolve from traditional data storage to support clustering, correlation, enrichment and labeling to support RCA and SIA. Inventory systems also need to be able to leverage structured data for forecasting, what-if analysis and predictions.
An example for such an innovative inventory solution is Nokia Unified Inventory, which is powered by a graph database to enable AI/ML use cases such as:
-
Proactive capacity management anticipates customer and service growth trends for better expenditure planning, while ensuring the network can accommodate future business growth
-
Network simulation and planning ensures alignment of future network scenarios with business requirements before actual deployment
-
Provisioning new services using the least utilized resources, decommissioning or migrating unused resources, identifying congested resources and planning for capacity increases or traffic diversion to underutilized resources ensures efficient network utilization
-
Network failure impact simulation identifies critical network resources based on the number of services utilizing them for priority monitoring and mitigates potential outages by preparing redundancy measures
Learn more about Nokia Unified Inventory and request a demo of our Digital Operations Center solution on our website.