Scaling up AI/ML for cellular radio access
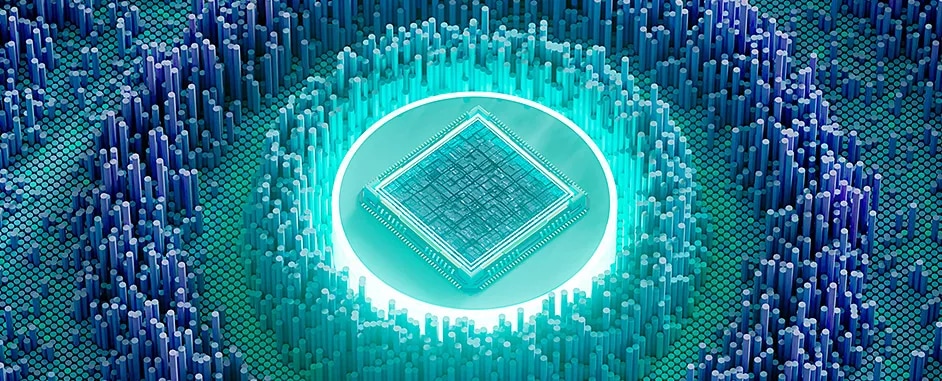
We are at the beginning of a revolution in cellular networks as AI/ML solutions for the air interface are poised to come to our phones and networks with the advent of 5G-Advanced. Nokia’s research results demonstrate substantial performance gains in terms of throughput (up to 30%), signaling overhead reduction (more than 30% reduction with the same performance), and other KPIs like energy efficiency, as explained in our previous blog. The technology revolution is real, but how do we get there in practice? Pure technology aside, the key to success is to develop solutions that are both economically and technically feasible to scale. In this blog, we will outline what it will take to succeed.
Figure 1. One-sided and two-sided AI/ML solutions in the mobile network air interface
AI/ML solutions for the air-interface can be categorized as shown in Figure 1. One-sided solutions assume the ML algorithm for a given feature is deployed at either the device side or the network side as, for example, in the beam prediction and positioning enhancement use cases. Two-sided solutions assume the ML algorithm is deployed in two parts and run jointly at both the device and network sides as, for example, in the device channel feedback ML-based compression use case. In this case, the device’s ML algorithm part compresses the channel state information (CSI) and the network’s ML algorithm part decompresses it to retrieve the CSI.
In order to ensure that different vendors’ ML implementations and algorithms for networks and devices work well together in all scenarios, standardization is required. Thus, Nokia is driving the work on a holistic standardized framework for 5G-Advanced in 3GPP, addressing both one-sided and two-sided solutions with control-plane signaling between the network and the device for the correct and controllable operation of the AI/ML solutions. This framework can be applied to any use case in the air interface and will be the foundation for the next generations of the AI-native air interface in 6G.
Specifically, lifecycle management (LCM) procedures will be introduced, addressing the different phases and requirements needed to enable an interoperable MLOps automation level in the radio – let’s call it Radio MLOps. This Radio MLOps should include procedures for data collection, development and testing, deployment, and operation and monitoring of ML solutions, as illustrated in Figure 2. Some of the procedures, like deployment, are mostly specific to the implementation. The framework provides device vendors, network vendors, and operators with the required tools for deploying and operating ML solutions in radio at scale — meaning hundreds and thousands of devices per cell with guaranteed interoperability.
The different stages in this figure also indicate one of the key benefits of ML and data-driven solutions, which is the possibility of continual improvement of the underlying ML model based on data and monitoring. This will ensure it can adapt to a changing environment or conditions.
Figure 2. Standardized elements of Radio MLOps enable AI at scale in the air interface
To make this work, data needs to be collected for training, for inference, and for performance monitoring. The framework needs to ensure that operators have control about how, what, when, and for which use cases data is collected, in compliance with local data and privacy regulations and reflecting our Nokia vision on Responsible AI. The challenge, mainly for training data, is to do this is in a scalable and interoperable way, so that different parties get the data needed in a controlled and efficient manner. To this end, the following principles should be followed for training data collection procedures:
-
Ensure user security and privacy
-
Make data accessible by the subscribed parties
-
Operator needs to be aware of and control data collection
-
Minimize additional air-interface traffic
-
Design for extensibility and future evolution.
One of the challenges of ML solutions in the radio compared to non-ML solutions are testability and performance requirements. How to ensure that solutions with ML components behave as intended in all circumstances and scenarios? Fallback mechanisms with a minimum guaranteed performance can be one option, especially if the ML solution is employed for high performance premium services or devices. Solutions without a (legacy) fallback, however, will need to fulfill the strongest requirements as well.
Consequently, Nokia believes that both pre- and post-deployment performance validation mechanisms are required. The ML solutions are tested and validated as part of the usual ML algorithm development process, based on 3GPP minimum requirement and test specifications. This could also include requirements for delay budgets for activation, deactivation and switching of ML functionalities to ensure robust operation of the LCM procedures.
After the ML solutions are deployed in the devices and/or the network nodes, in-field, post-deployment monitoring and validation mechanisms are used to ensure reliable operations in real network deployments. Performance monitoring of ML functionalities is also needed to ensure robust operation in the field. It will be triggered whenever a performance degradation is detected, for example, because of data drift due to changing radio environments. The measured KPIs are use-case-specific and are collected along the already specified performance KPIs for existing non-ML solutions.
Going from ML-enabled to AI-native
MLOps enables organizations to develop, deploy and maintain machine learning models at scale and with greater efficiency. Additionally, AI/ML-based solutions have the potential to further extend the boundaries of performance of the air interface. We have outlined what it takes to deploy AI/ML solutions in the air interface at scale – a scalable and inter-operable AI/ML framework, future-proof, and ready for additional use cases in 6G and beyond. To this end, the standardization work in 5G-Advanced on ML enablers will pave the way for AI-native 6G, where AI and ML are considered from the start as key design principles of the system.