Smarter broadband anomaly detection with AI
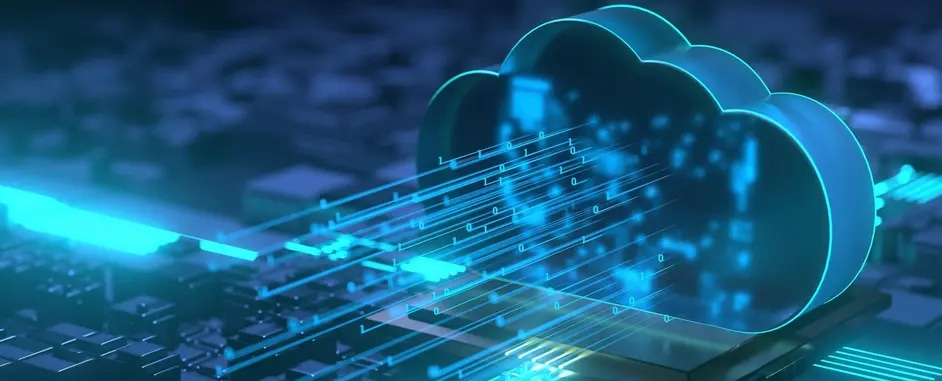
In this blog series, we’re looking at how Artificial Intelligence (AI) and Machine Learning (ML) are transforming fiber broadband network operations. After an overview in the first blog on how the addition of AI decision elements helps to create autonomous networks, we moved on to specific use cases, starting with AI-assistance to humans and, next in this blog, anomaly detection.
In today's modern broadband network environments, performance data is abundantly available. Streamed from network devices, a vast amount of information is constantly being created and stored. However, the challenge lies not in the data itself, but in effectively processing, identifying, and acting upon it. This task can be daunting.
Within all this data lies a wealth of insights for improving network operations. Chief among them is detect or predict issues before they become service affecting. Identifying and addressing anomalies promptly and with high accuracy is essential for maintaining network reliability and customer satisfaction. However, the traditional anomaly detection methods often involve a lot of manual effort and expertise to set the appropriate thresholds and rules. This can be time-consuming, error-prone, and may not actually be able to detect subtle or complex anomalies.
AI/ML offers solutions that can significantly improve anomaly detection. This involves analyzing data collected from the network to recognize patterns, predict trends, correlate information, and identify unusual events that may indicate problems. It relies on defining what “normal” looks like so that deviations from that norm can be detected. While the concept seems straightforward, networks are dynamic, demonstrating constantly evolving "normal" behavior. AI and ML techniques can adapt to these changing conditions more effectively than traditional methods. They offer more flexible options, using statistical or model-based techniques, ranging from simple moving averages to time series models that can account for daily variations, seasonality and trends.
In optical signal power monitoring, for example, AI/ML techniques can detect anomalies that represent fiber bends, dirty connectors, or disconnected fibers, allowing for swift identification of physical network problems. AI can also analyze performance metrics like board temperature and CPU load to identify potential hardware failures before they occur, enabling proactive maintenance.
Multivariate anomaly detection using multi-KPI correlation or clustering techniques proves valuable for identifying degrading performance. By simultaneously analyzing multiple performance indicators, this approach can detect subtle signs of degradation that might be missed by simpler methods.
Statistical methods powered by AI can also analyze a user's bandwidth consumption over time to identify various types of bottlenecks in the network. This granular understanding of bandwidth usage patterns allows for more targeted network optimizations. Lastly, AI excels in alarm correlation, where it can analyze and correlate equipment alarms for root cause analysis and fault localization. This capability is particularly useful for detecting issues like rogue ONTs or cut fibers, streamlining the troubleshooting process and reducing time to resolution for network issues.
The following diagram illustrates a number of these anomaly detection scenarios, for which AI/ML is perfectly suited.
AI/ML solutions scale efficiently as networks grow, automate detection processes, and enable proactive maintenance. This leads to reduced operational expenses, minimized downtime, and improved service quality through faster problem detection and resolution.
As broadband networks continue to grow in complexity, broadband providers need to evolve their management approaches. By implementing AI/ML technologies, operators can better address current challenges and prepare for future network demands.
Watch out for the next blog in this series and, in the meantime, download this white paper to learn more. Also, why not check out the Nokia Altiplano Access Controller, the domain controller that brings sophisticated intelligence into the fixed access domain, which was awarded the #1 network automation platform for broadband access networks worldwide in 2024.