Your network is wasting energy and Artificial intelligence can change that
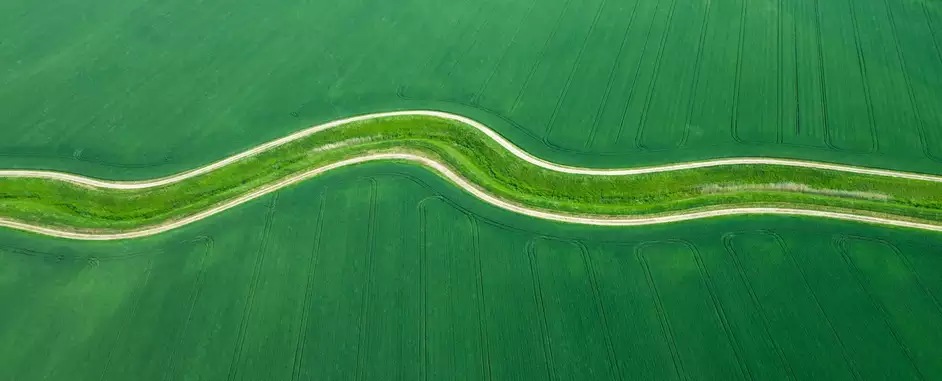
Sustainability is no longer optional
Telecommunications may account for just 1% of global electricity consumption — but that 1% adds up to a significant amount of CO2 emissions and is a major cost for communication service providers (CSPs). While CSPs are eager to reduce their power consumption, the challenge is to do it without compromising network performance, customer satisfaction or the bottom line.
The goal of bringing usage down is hampered partly by the fact that most of the world’s energy still comes from non-renewable greenhouse gas (GHG)-emitting sources, and partly because the world is only becoming more and more connectivity-dependent. And while that connectivity itself contributes to GHG reductions in other sectors (especially with the 90% higher energy efficiency of 5G), network power consumption is bound to rise just by virtue of demand.
Yet CSPs are under pressure from customers, regulators and governments to take action. Conserving energy is no longer lip service: it’s a business imperative. Investors are also taking a stand: according to Deutsche Bank, on the S&P 500, companies with low GHG emissions are rated more than 15% higher than those with above-average CO2 emissions, and even higher than companies that don’t publish data on their emissions.
Start by reducing unproductive energy
The key question is how to cut the largest possible chunk of energy consumption at the fastest possible pace. For most CSPs, the radio access network (RAN) accounts for some 80% of all mobile network energy consumption — and only 15% of that is used to transmit data. The other 85% goes into non-revenue generating consumption such as heating and cooling systems, lighting, uninterruptible and other power supplies, and running idle resources.
All that power not only drives up emissions but also costs CSPs in a big way, totaling anywhere from 10% to as much as 40% of their network expenses, making the RAN the most logical first target energy efficiency measures.
Truly dynamic power management shrinks the carbon footprint immediately
Becoming energy efficient is about stopping energy waste which happens on a massive scale in everyday operations, both at active radio components and auxiliary passive equipment.
A simple way to reduce energy management is to schedule equipment shutdowns. But because those aren’t based on “live” real-world conditions, they risk leaving the network short-handed. Just-in-time waking is hard to achieve with static or rules-based methods, usually requiring wide wake windows or extensive use of stand-by mode to prevent a poor customer experience when traffic starts increasing again.
Instead, there are two key strategies to reduce network energy use: dynamic network shutdowns and full-site power management.
Dynamically shutting down unused network elements during low-traffic periods can save a significant amount of energy. Artificial intelligence (AI) is the key to taking full advantage of this strategy and maximizing savings. Drawing on all sorts of data, AI can perform precise predictions to perfectly balance energy savings, network performance and customer experience requirements.
Experience has shown that using AI for dynamic shutdowns can extend sleep times by several hours. And AI can increase energy savings by another 50% by eliminating the need to keep resources on stand-by.
Energy management must cover passive equipment
But dynamic shutdowns only account for network elements. At most radio sites, 50% of energy consumption goes to auxiliary components such as fans, cooling systems, lighting and other power supplies. To make sure those also perform as efficiently as they should, AI-powered energy consumption management has to cover both active radio and passive equipment — benchmarking energy trends to spot performance anomalies in historically “invisible” passive equipment that could be draining energy and might need to be reconfigured or replaced.
Implementing AI-based energy management can reduce energy costs and carbon footprint by 20–30% with no negative impact on performance or customer experience.
The best news is that, because of its software nature, AI-based energy efficiency can be deployed in just a few weeks without major upfront investment — especially thanks to outcome-based business models, which enable you to pay your vendor only for the outcomes you actually achieve. Implementing it over a public cloud can make it even faster by easing the processing and analysis of the large volume and velocity of network data.
So for any CSPs out there who have been waiting for the right solution to come along and help them meet their sustainability goals with energy savings: the wait is over.