On the road to AI-native networks
Technology Vision
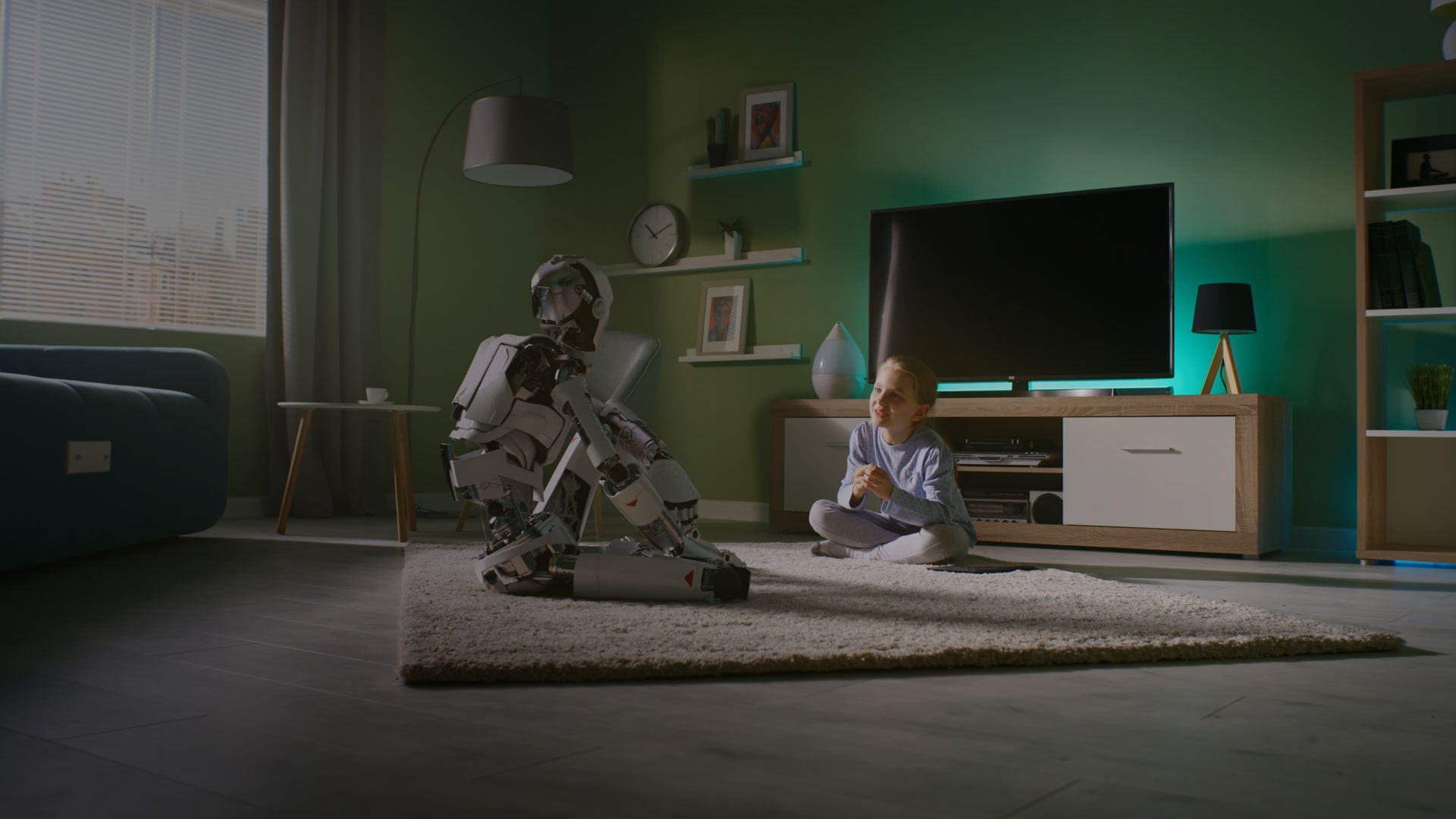
While the roots of Artificial Intelligence (AI) go back to the middle of the last century, it has only recently begun to capture the full imagination of the public. AI today is only at its infancy in terms of its use and potential across various industries. As connectivity is key for most of these services, network suppliers and connectivity providers are at the center of questions on what networks can do for AI and what AI can do for networks. Please join us on the road to AI-native networks.
The birth of Artificial Intelligence: The term "Artificial Intelligence" was coined in 1955 by computer scientist John McCarthy who along with Claude Shannon from our Bell Labs and two other co-authors wrote a paper proposing a workshop on AI that was held the following year. This 1956 workshop became the first AI conference, marking the formal inception of the field. Over the decades, AI experienced various phases of progress and setbacks.
Enlightening moment for AI: The most recent significant milestone occurred in 2017 when Google introduced the self-attention mechanism, which laid the foundation for the transformer architecture. This design is now integral to the most popular large language models, including OpenAI’s GPT, Anthropic’s Claude, Meta’s Llama or Google’s Gemini.
ChatGPT, a chatbot service based on OpenAI’s GPT, amazed people around the globe in 2022 with the release of its 3.5 version and its advanced capabilities in understanding and generating human-like text. It quickly became a sensation, achieving the fastest adoption rate of any service in history. Users were astounded by its ability to engage in coherent and contextually relevant conversations, perform complex tasks, and provide valuable insights across various domains. The success of ChatGPT led to a surge in funding for AI research and development, marking the beginning of a new era of AI applications and ambitions.
Beyond GenAI: While ChatGPT leverages transformer-based Large Language Model (LLM) technology, it is important to recognize that there are many other types of AI models. These include regression models, which predict continuous outcomes, clustering models, which group data points based on similarity, classification models, which categorize data into predefined classes, reasoning models, which simulate logical decision-making processes starting with expert systems to current evolving LLMs and neuro-symbolic algorithms, and models designed for computer vision that are especially effective in image and video recognition tasks such as Convolutional Neural Network (CNN) and diffusion models. Each type of AI model serves different purposes and applications, contributing to the diverse and multifaceted landscape of artificial intelligence applications.
AI's impact on the world: This mix of AI models is starting to impact all areas of our world. We may not always see it, but the technologies we use in our daily lives are quickly adopting AI because it brings undeniable gains. Just to mention a few, AI is influencing communication networks, semiconductors, security and privacy, our sustainability efforts, and even quantum technologies while at the same time, these areas impact AI as well. As with any powerful tool, AI offers significant benefits, but it also presents challenges that need to be addressed. By harnessing AI responsibly, we can maximize its positive impact while mitigating potential risks.
This new wave of AI adoption holds immense potential to revolutionize how we interact and connect within the interwoven realms of the human, digital and physical worlds. By seamlessly bridging these domains, AI promises to create interactions that are more natural, valuable and contextually relevant. Enabling a more intuitive understanding between humans and the diverse array of machines, robots and agents that populate our increasingly interconnected world will make these interactions feel more human to us.
These interactions can only be made possible via communication networks, so let's see how AI is influencing future networks.
How AI is relevant for future networks?
The future of communication networks lies in the integration of artificial intelligence, creating a symbiotic relationship that will drive innovation and unlock unprecedented possibilities across industries. This integration will enable unprecedented levels of connectivity, speed, and intelligence across networks. For instance, 5G and future 6G networks will provide the backbone for a wide range of intelligent devices, while AI will optimize network performance and security. In turn, the massive amounts of data generated by these connected devices will fuel advancements in AI and cloud services.
AI will be …
In networks:
AI-native functions replace traditionally designed features.
Over networks:
Includes over-the-top AI applications plus apps recommended by AI engines.
Using networks:
3rd party developers create AI/non-AI apps using network APIs which could be recommended by coding assistants.
For networks:
AI apps for managing networks and AI apps for CSPs & Enterprises to offer to subscribers, employees and customers.
In networks, we will witness artificial intelligence (AI) revolutionizing the communication network architecture across all domains, including Radio Access Network (RAN), Core, Fixed Access, Transport, Orchestration and Management and user equipment (UE). In addition, AI's influence will extend beyond individual domains, with use cases impacting multiple areas simultaneously or independently, creating a truly transformative network experience.
AI everywhere:
- Energy efficiency
- Quality of service (QoS)
- Network optimization
- Resource scheduling
- Load balancing
- Anomaly detection
- Device management
- Intent-based autonomy
Orchestration & Management with AI: While automation in network management and orchestration has been a top priority for several years, full autonomous systems that operate with minimal to no human intervention (level 5 automation) will be possible only with extended AI capabilities. These advanced networks will self-manage all operations, from routine maintenance to complex problem-solving. They will automatically detect and repair faults, optimize traffic flows in real-time and instantly identify and mitigate security threats. Interaction with human operators will change. Instead of requiring intricate knowledge of parameters and manual configurations, communications will be based purely on human intent. This intuitive approach will simplify network management, making advanced technology accessible to a broader range of users that could also include external systems and 3rd party companies.
Cross domain benefits of AI: As one example, energy efficiency will see gains through an end-to-end approach powered by AI and machine learning. These systems will accurately predict traffic patterns, enabling proactive optimization across the entire network infrastructure. By analyzing data on traffic, node loads and user behavior, networks will dynamically optimize power states and resource allocation. This intelligent management will manifest in various ways: dynamic power state transitions in Radio Access Networks (RAN), optimized route balancing in transport networks and efficient bandwidth scaling in user devices. This holistic approach not only reduces energy consumption but also enhances overall network performance to achieve more sustainable and responsive communication systems.
Where are we on the road to AI-native networks?
The evolution to AI-enabled networks: AI/ML is not new for communications networks, with applications in mobile networks dating back to 2009. Self-organizing networks (SON), introduced with LTE in 3GPP Release 8 in 2009, used AI/ML to automate network planning, configuration and management.
This marked the beginning of the evolution toward what we call "AI-enabled networks," where artificial intelligence plays a role in a small number of network functions. This AI integration is primarily focused on the management domain, such as Self-Organizing Networks (SON) , or on data and analytics, as seen in the Network Data Analytics Function (NWDAF) initially defined in 5G networks for core domain. While these AI use cases offer valuable insights and automation, they are often implemented in silos, with limited collaboration and interoperability between different AI systems and domains.
The future of AI-native networks: Currently AI-enabled networks are optimizing network performance, detect and prevent cyber attacks, and enhance customer experiences just to mention a few applications. They have already surpassed traditionally designed networks in terms of performance/TCO. However, after several decades of evolution in communication networks, we are encountering limits in various areas, such as the increasing complexity of managing and optimizing networks with thousands of parameters, and the approaching limits in spectral efficiency. We believe that future “AI-native” networks will break these barriers in networking and can lead to major innovation "S-curves" in terms of what networks can achieve regarding performance, monetization and cost efficiency .
This will all be possible as AI capabilities are extended to include reasoning, planning and function calling resulting in AI agents becoming more autonomous. These agents can make decisions and act without direct human intervention. Recent advancements in Large Action Models (LAMs) have significantly enhanced AI agent tasks, excelling in function calling through external processes and extending the functionality of LLMs beyond generating static text and images.
What do we mean by AI native networks?
What does it mean for a network to be AI-native? In an AI-native network architecture, a significant portion of network functions (NFs) on both the control and user planes are powered by AI. This advanced design relies on a robust ecosystem of "AI-native support" services, including AI orchestration, model management and performance monitoring, to ensure seamless integration and optimal performance of AI-based NFs.
An AI-native network requires interoperation, collaboration and sometimes integration between AI-based NFs. This involves a holistic approach to network design and optimization, leveraging the power of AI to deliver enhanced user experiences and superior network efficiency.
While the full realization of an AI-native network is envisioned for the 6G era, the foundation for this transformation will be laid in the coming years, paving the way for a future where AI is deeply embedded in every aspect of the communication network.
AI-native trials in Network Functions (NF) are demonstrating remarkable potential for transforming telecommunications. In a collaboration between NTT Corporation, Nokia and SK Telecom Co., Ltd., AI-powered solutions have achieved up to 18% improvement in indoor communication speeds compared to conventional methods, showcasing AI's ability to optimize network performance in challenging environments. Nokia Bell Labs has also announced an industry-first research breakthrough called Natural-Language Networks, which will allow networks to be operated through plain speech or text prompts. These intelligent networks will understand user intentions and act upon them autonomously, paving the way for more intuitive and accessible network interactions.
True AI native network will consists of:
- A large number of AI-based network functions (NFs) on the control and user plane
- AI-based NFs supported through common “AI-native support” services
- Stronger collaboration / integration between AI-based NFs
The transition to AI-native networks and beyond
Initially, AI-native network functions will be added on top of existing networks, enhancing their capabilities and gradually integrating AI-native features into the network infrastructure. New AI-native architecture and hardware will be deployed in areas where further network and energy enhancements are not otherwise possible. AI-native network capabilities will initially emerge in specific areas and components, before eventually becoming the standard throughout networks. 6G networks are designed to be AI-native from the beginning, but the development and deployment of AI-native networks will be a long-term process, requiring significant research and development efforts.
The ultimate goal is what we refer to as "Self-evolving networks." In these networks, Network Functions (NFs) and controllers are not only AI-based but also function as self-evolving AI agents. This means that the network autonomously evolves and develops new capabilities over time, continually enhancing its performance and adaptability without human intervention.
Critical success factors to AI deployment
AI-native networks will be developed over the coming decades, coexisting with the current infrastructure. The path to achieving the target network will be shaped not only by advancements in AI technology but also by progress in several related fields.
Key areas of focus include:
Performance and Computational
The demands of current AI models
Current AI models are highly demanding in terms of processing power and energy consumption. To address these challenges, further development is needed in several areas, including the advancement of small language models, split processing and the commoditization of AI hardware.
Progress and innovations in AI models and architectures
Progress is being made on all fronts. Some small language models operating on devices and at the edge are already achieving performance levels comparable to GPT 3.5 on the MMLU benchmark. Various companies and academic institutions are exploring new AI model architectures aimed at increasing efficiency and reducing power and computational intensity, moving beyond the current state-of-the-art transformer models.
Advancements in AI hardware and emerging technologies
On the hardware side, as Moore's Law reaches its limits and Dennard scaling comes to an end, there is growing interest within the computing community to explore alternative, more energy-efficient technologies that can sustain ongoing improvements in performance. Trends such as in-memory computing and multi-die architectures are already emerging, but this is a dynamic field that requires much more research to fully power the AI revolution. Active fields of exploration include GPU accelerators, Quantum AI computing, Neuromorphic Computing, and on-device/edge processing. These technologies hold promise for significantly enhancing the efficiency and performance of AI systems.
Security and Privacy
Standardization and Specification
Uncertainty and Regulation
The demands of current AI models
Current AI models are highly demanding in terms of processing power and energy consumption. To address these challenges, further development is needed in several areas, including the advancement of small language models, split processing and the commoditization of AI hardware.
Progress and innovations in AI models and architectures
Progress is being made on all fronts. Some small language models operating on devices and at the edge are already achieving performance levels comparable to GPT 3.5 on the MMLU benchmark. Various companies and academic institutions are exploring new AI model architectures aimed at increasing efficiency and reducing power and computational intensity, moving beyond the current state-of-the-art transformer models.
Advancements in AI hardware and emerging technologies
On the hardware side, as Moore's Law reaches its limits and Dennard scaling comes to an end, there is growing interest within the computing community to explore alternative, more energy-efficient technologies that can sustain ongoing improvements in performance. Trends such as in-memory computing and multi-die architectures are already emerging, but this is a dynamic field that requires much more research to fully power the AI revolution. Active fields of exploration include GPU accelerators, Quantum AI computing, Neuromorphic Computing, and on-device/edge processing. These technologies hold promise for significantly enhancing the efficiency and performance of AI systems.
AI in cybersecurity
AI, security and privacy need to be considered in three dimensions: AI used to protect, AI to be protected and AI used for attacks.
AI-powered cybersecurity: Benefits and challenges
Implementing AI across the entire cybersecurity lifecycle brings substantial improvements, such as adapting cyber defense strategies to the constantly evolving threat landscape and automating repetitive cybersecurity tasks. On the other hand, AI lowers the entry barrier for novice cybercriminals, leading to an increase in cyberattacks. AI enhances tactics, techniques and procedures (TTP), making social engineering attacks harder to detect.
Risks and vulnerabilities of AI systems
AI-enabled systems pose risks of malfunctioning and privacy violations due to biased decision-making, untrusted data or the exploitation of AI-specific security vulnerabilities. These systems are susceptible to various vulnerabilities, including data poisoning, adversarial attacks and model inversion. Data poisoning involves injecting malicious data to corrupt the training process, while adversarial attacks manipulate inferencing inputs to deceive the AI. Model inversion allows attackers to infer sensitive information from the model. In addition, biases in training data can lead to unfair or inaccurate outcomes, and the complexity of AI models can make them difficult to interpret and secure.
Mitigating risks and ensuring Responsible AI
Various measures - including ensuring data security, integrity and privacy, maintaining the quality of training and (when possible) inferencing data, and addressing biases in AI models together with standardization and regulations - are taken to mitigate the risk.
Optimizing networks and devices for large-scale AI
Developing large-scale AI systems necessitates changes in the design, operation and optimization of networks and devices.
The journey towards AI-native 6G in mobile networks
In mobile networks, the journey toward AI-native 6G has already begun with 5G-Advanced (Release 18) studies and the standardization of multiple features. These findings indicate necessary changes in areas such as Layer 2, testability, Quality of Service (QoS), and reliability, among others.
Future standardization and AI-native networks
In the coming years, with the advent of 3GPP Release 21 and subsequent releases, we will witness further standardization enabling AI-native networks.
The need for AI governance
The rapid development of Artificial Intelligence (AI) has sparked widespread concern about its potential risks. Recognizing this, various international organizations, including the United Nations, OECD, G20, G7 and the Council of Europe, have initiated efforts to govern AI, each with distinct goals, scopes and strategies. These initiatives reflect the diverse political, economic and ethical concerns surrounding AI technologies.
Emerging approaches to AI legislation
Governments worldwide are increasingly emphasizing the need for legal frameworks to address the risks associated with AI. Three primary approaches are emerging:
- Targeted legislation: Focusing on high-risk AI systems, with governments proposing legislation to mitigate specific risks, such as bias, discrimination and privacy violations.
- Promoting principles and responsible use: Encouraging the adoption of ethical principles and responsible AI practices through guidelines and voluntary standards.
- Regulation of specific technologies: Developing rules for emerging technologies like deep synthesis and generative AI systems, which are currently under debate and adoption.
Navigating uncertainties and ensuring Responsible AI development
Navigating the uncertainties surrounding AI development and regulation will be crucial to creating a stable and compliant network environment. Collaboration between governments, industry and academia is essential to ensure that AI technologies are developed and deployed responsibly, maximizing their benefits while mitigating potential risks.
How to prepare
The journey towards AI-native networks has already begun. This transformation extends beyond automating networks and minimizing downtime to encompassing the evolution of companies to becoming AI-native, ensuring responsible AI practices and leveraging AI for the new API economy. To learn more about accelerating AI to advance both business and society, visit our Technology Strategy Artificial Intelligence page.

AI technology strategy
The AI revolution is underway and will profoundly change the way people live and work.