In future networks, 6G radios will learn from one another
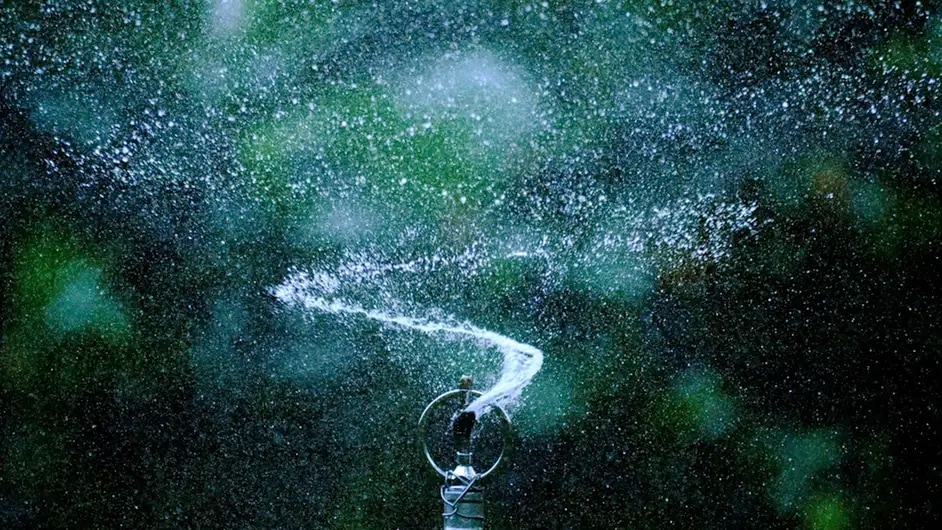
The quest for artificial intelligence that mimics the amazing capabilities of the human brain began soon after the invention of the computer. After several cycles of exuberance and disappointment, we are now in the midst of an era in which machine learning is being broadly applied to substantially enhance the state of the art in a variety of different areas: image recognition and classification, natural language processing, and robotic systems, to name just a few.
The beauty of artificial intelligence and machine learning (AI/ML) is that the basic approach of data-driven, trained systems easily extends to solving problems in multiple domains. At Nokia Bell Labs we began wondering several years ago if we could apply those same problem-solving AI/ML techniques to wireless systems. What if we could engineer radios with the ability to adapt their signaling schemes and protocols to achieve the best performance for any given environment, in any given situation, unfettered by pre-defined parameters?
We’ve made good progress toward that goal, and we expect that a dynamic AI/ML-defined native air interface will be a key component of 6G networking in the future. This would at the very least revolutionize the way we standardize and productize wireless systems. But eventually these interfaces could give radios the ability to learn from one other and their environments. Rather than engineers telling network nodes of the network how they can communicate, those nodes could determine for themselves – choosing from millions of possible configurations – the best possible to way to communicate.
How we envision the role of AI/ML in the 6G air interface is the subject of a just published Nokia Bell Labs white paper, but we’ll explore the implications of this technological shift in more detail below.
Embedding AI/ML deeper in the network
ML is not something completely new to wireless. For example, self-optimizing networks (SON) make extensive use of it. The SON approach to network management has always been based on applying traditional machine-learning techniques to large volumes of changing operational data to derive the most optimal network parameter settings over time. Meanwhile, deep learning is being applied to solve SON and radio resource management (RRM) problems, such as load balancing, carrier aggregation, handover optimization and anomaly detection.
An AI/ML-based air interface would bring that intelligence much deeper into the network, applying it to real-time signal processing tasks in the transmitter and receiver. These are tasks traditionally solved through sound mathematical modeling driving near-optimal algorithms – techniques that have been perfected by human designers over five generations of mobile wireless systems. Signal transmission and reception is at the heart of these systems and has been steadily improving over time. But by introducing AI/ML to the process, we can push the boundaries even further to enable a self-optimizing air interface that is perfectly adapted to any channel, hardware and application sitting on top of it.
After a several years of investigating various applications of AI/ML to physical-layer and medium-access-layer (MAC) processing, we are beginning to see some promise. We have developed learned receiver blocks for 5G that consistently outperform traditional baselines. By jointly optimizing transmitter and receiver, we’ve seen additional throughput gains. And we’ve made rapid progress in ML hardware accelerators that will make native AI/ML air interfaces not only practical but also very energy efficient.
Even though wireless capacity is already close to the fundamental theoretical limits as defined by Bell Labs’ own Claude Shannon, every expert in the field knows there is a big gap between real-world performance and theoretical performance. AI/ML may not be able to extend Shannon’s Limit, but it could significantly bridge the gap between theory and reality by making the most efficient connection possible in any scenario.
First, it could dynamically learn and set up bespoke waveforms, constellations, and pilot signals that make more efficient use of available spectrum, resulting in better performance in certain scenarios such as higher mobility MIMO transmissions. Second, on the MAC layer, AI/ML could customize signaling and access schemes, which could, for example, seamlessly switch between contention-based or scheduled access adapting to the service needs automatically. Third, a learned air interface could embrace hardware non-linearities and limitations and fully adapt to them whatever the target platform is. Fourth, AI/ML could choose among all the myriad of parameters in a radio network in ways no human can optimally accomplish.
What does this mean for future 6G networks? It means that networks could eventually adapt to any data source, application or use case. This flexibility would be particularly powerful in private networks, such as industrial communications systems. The radios in a factory, for instance, might be configured to support industrial sensors at one moment, but in the next they could reconfigure to support robotic orchestration or video surveillance – moving to different spectrum bands, reconfiguring the number of signal paths and changing modulation schemes as the specific application demands. What’s more, by learning from their environments, those radios could adapt to millions of different scenarios that engineers might not anticipate. Even if they could define every single one of those scenarios, the industry can’t standardize millions of different configurations. A flexible interface that auto-adapts could very well change the face of network optimization.
Finally, a learned AI/ML air interface could transform how R&D is done. If we can truly implement all transmitter and receiver signal processing functions through machine learning, then we can fundamentally alter the way algorithms are designed. Instead of separating the receiver functions into multiple separate algorithms, each of which is then implemented as software on special purpose hardware, design would focus on partitioning functions and selecting the best machine learning model architectures for each function. The subsequent implementation on hardware as well as training would be significantly automated using various tools.
The larger 6G context
We feel that the AI/ML-based air-interface could be a transformative element of future 6G networks, one of six key components we’ve outlined in the Nokia Bell Labs white paper Communications in the 6G era. In that era, the inexorable demand for higher performance and higher capacities will require exploiting ever-higher bands, wider bandwidth signals, new solutions for joint communication and sensing, and new approaches to security and trust.
These technologies will encourage a 6G design that is not only optimized for communication but also to help its users perceive and understand the physical world, thus augmenting human abilities in the most intuitive ways. To achieve that goal, we will need to take advantage of every tool at our disposal, so it only makes sense that we exploit the rapid advances in AI/ML to create the most flexible, adaptive networks possible.
The full white paper is available for download at the following link.